Creating a Custom Image in a Notebook Instance Using the Image Saving Function
Description
This section describes how to import a local model package to ModelArts notebook for debugging and saving, and then deploy the saved image for inference. This case applies only to Huawei Cloud CN North-Beijing4 and CN East-Shanghai1 regions.
The procedure is as follows:
Step1 Copying a Model Package in a Notebook Instance
- Log in to the ModelArts console. In the navigation pane on the left, choose Development Workspace > Notebook.
- Click Create in the upper right corner. On the Create Notebook page, configure the parameters.
- Configure basic information of the notebook instance, including its name, description, and auto stop status.
- Select an image and configure resource specifications for the instance.
- Image: Select image tensorflow_2.1-cuda_10.1-cudnn7-ubuntu_18.04 or pytorch1.8-cuda10.2-cudnn7-ubuntu18.04. For details about the images, see Engine Version 1: tensorflow_2.1.0-cuda_10.1-py_3.7-ubuntu_18.04-x86_64 and Engine Version 1: pytorch_1.8.0-cuda_10.2-py_3.7-ubuntu_18.04-x86_64.
- Resource Type: Select a public resource pool or a dedicated resource pool. A public resource pool is used as an example.
- Type: GPU is recommended.
- Flavor: GP Tnt004 is recommended.
- Click Next. Confirm the information and click Submit.
Switch to the notebook instance list. The notebook instance is being created. It will take several minutes
- before its status changes to Running. Then, locate the notebook in the list and click Open in the Operation column. The JupyterLab Launcher page is displayed.
Figure 1 JupyterLab Launcher
- Click
to upload the model package file to the notebook instance. The default working directory of the instance is /home/ma-user/work/. Prepare the model package file. For details, see Sample Model Package File.
Figure 2 Uploading a model package - Start the Terminal. Decompress model.zip and delete it.
# Decompress the ZIP file. unzip model.zip
Figure 3 Decompressing model.zip on the Terminal - In the Terminal tab, run the copy command.
cp -rf model/* /home/ma-user/infer/model/1
Check whether the image file is copied.
cd /home/ma-user/infer/model/1
ll
Figure 4 Image file copied
Sample Model Package File
A model file in the model package file model.zip must be prepared by yourself. The following uses a handwritten digit recognition model as an example.
The inference script file customize_service.py must be available in the model directory for model pre-processing and post-processing.

For details about the inference script customize_service.py, see Specifications for Writing a Model Inference Code File customize_service.py.
The content of the customize_service.py file used in this case is as follows:
import logging import threading import numpy as np import tensorflow as tf from PIL import Image from model_service.tfserving_model_service import TfServingBaseService class mnist_service(TfServingBaseService): def __init__(self, model_name, model_path): self.model_name = model_name self.model_path = model_path self.model = None self.predict = None # Load the model in saved_model format in non-blocking mode to prevent blocking timeout. thread = threading.Thread(target=self.load_model) thread.start() def load_model(self): # Load the model in saved_model format. self.model = tf.saved_model.load(self.model_path) signature_defs = self.model.signatures.keys() signature = [] # only one signature allowed for signature_def in signature_defs: signature.append(signature_def) if len(signature) == 1: model_signature = signature[0] else: logging.warning("signatures more than one, use serving_default signature from %s", signature) model_signature = tf.saved_model.DEFAULT_SERVING_SIGNATURE_DEF_KEY self.predict = self.model.signatures[model_signature] def _preprocess(self, data): images = [] for k, v in data.items(): for file_name, file_content in v.items(): image1 = Image.open(file_content) image1 = np.array(image1, dtype=np.float32) image1.resize((28, 28, 1)) images.append(image1) images = tf.convert_to_tensor(images, dtype=tf.dtypes.float32) preprocessed_data = images return preprocessed_data def _inference(self, data): return self.predict(data) def _postprocess(self, data): return { "result": int(data["output"].numpy()[0].argmax()) }
Step2 Debugging a Model in a Notebook Instance
- In a new Terminal tab, go to the /home/ma-user/infer/ directory, run the run.sh script, and predict the model. In a base image, run.sh is used as the boot script by default. The start command is as follows:
sh run.sh
Figure 6 Running the boot script - Upload an image with a handwritten digit to the notebook instance for prediction.
Figure 7 Handwritten digitFigure 8 Uploading an image for prediction
- Open a new Terminal and run the following command for prediction:
curl -kv -F 'images=@/home/ma-user/work/test.png' -X POST http://127.0.0.1:8080/
Figure 9 PredictionIf the model file or inference script file is modified during debugging, restart the run.sh script. To do so, run the following command to stop Nginx and then run the run.sh script:
# Obtain the Nginx process. ps -ef |grep nginx # Stop all Nginx-related processes. kill -9 {Process ID} # Execute run.sh. sh run.sh
You can also run the pkill nginx command to stop all Nginx processes.
# Stop all Nginx processes. pkill nginx # Execute run.sh. sh run.sh
Figure 10 Restarting run.sh
Step3 Saving an Image in a Notebook Instance
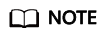
A running notebook instance must be available.
- Locate the target notebook instance in the list and choose More > Save Image in the Operation column.
- In the displayed Save Image dialog box, configure the parameters. Then, click OK.
Choose an organization from the Organization drop-down list. If no organization is available, click Create on the right to create one.
Users in an organization can share all images in the organization.
- The image will be saved as a snapshot, which will take about 5 minutes. During this period of time, do not perform any operations on the instance. (You can still perform operations on the accessed JupyterLab page and local IDE.)
The time required for saving an image as a snapshot will be counted in the instance running duration. If the instance running duration expires before the snapshot is saved, saving the image will fail.
- After the image is saved, the instance status changes to Running. View the image on the Image Management page.
- Click the image name to view its details.
Step4 Using the Saved Image for Inference Deployment
Import the custom image debugged in Step2 Debugging a Model in a Notebook Instance to models and deploy it as a real-time service.
- Log in to the ModelArts console. In the navigation pane on the left, choose Model Management. On the displayed, click Create Model.
- Configure the parameters, as shown in Figure 11.
- Meta Model Source: Select Container image.
- Container Image Path: Click
to select an image file. For details about the path, see the SWR address in 5.
- Container API: Select HTTPS.
- host: Set to 8443.
- Deployment Type: Select Real-Time Services.
- Enter the boot command.
sh /home/ma-user/infer/run.sh
- Enable APIs, edit the API, and click Save. Specify a file as the input. The following shows a code example.
Figure 12 API definition
The API definition is as follows:
[{ "url": "/", "method": "post", "request": { "Content-type": "multipart/form-data", "data": { "type": "object", "properties": { "images": { "type": "file" } } } }, "response": { "Content-type": "applicaton/json", "data": { "type": "object", "properties": { "result": { "type": "integer" } } } } }]
After enabling this function, you can edit RESTful APIs to define the input and output formats of a model.
- Configure APIs during model creation. The system will automatically identify the prediction type after the created model is deployed.
- Do not configure APIs during model creation. You will be required to select a request type for prediction after the created model is deployed. The request type can be application/json or multipart/form-data. Select a proper type based on the meta model.
- If you select application/json, enter code for text prediction.
- If you select multipart/form-data, configure the request parameter. The request parameter value is the KEY value in the Body tab when GUI-based software (Postman as an example) is used for prediction. It is also the parameter name in the prediction request sent by running the cURL command.
- After the APIs are configured, click Create now. Wait until the model runs properly.
- Click the triangle on the left of the created model name to expand the version list. Choose Deploy > Real-Time Services in the Operation column. The page for deploying the AI application as a real-time service is displayed.
- On the Deploy page, set key parameters as follows:
Name: Enter a custom real-time service name or use the default name.
Resource Pool: Select a public resource pool.
Model Source and Model and Version: The model and version are automatically selected.
Specifications: Select specifications with limited time offer.
Retain default settings for other parameters.
If free specifications have been sold out, use charged CPU specifications instead. Resource fees are displayed on the GUI.
- After configuring the parameters, click Next, confirm parameter settings, and click Submit.
- In the navigation pane on the left, choose Service Deployment > Real-Time Services. When the service status changes to Running, the service is deployed. Click Predict in the Operation column. The Prediction page on the service details page is displayed. Upload an image for prediction.
Feedback
Was this page helpful?
Provide feedbackThank you very much for your feedback. We will continue working to improve the documentation.See the reply and handling status in My Cloud VOC.
For any further questions, feel free to contact us through the chatbot.
Chatbot