Example of Starting PyTorch DDP Training Based on a Training Job
This topic describes three methods of using a training job to start PyTorch DDP training and provides their sample code.
- Use PyTorch preset images and run the mp.spawn command.
- Use custom images.
- Run the torch.distributed.launch command.
- Run the torch.distributed.run command.
Creating a Training Job
- Method 1: Use the preset PyTorch framework and run the mp.spawn command to start a training job.
For details about parameters for creating a training job, see Table 1.
Table 1 Creating a training job (preset framework) Parameter
Description
Algorithm Type
Select Custom algorithm.
Boot Mode
Choose Preset image and set AI Engine to PyTorch. Configure the PyTorch version based on your training requirements.
Code Directory
Select the path where the training code folder is stored in the OBS bucket, for example, obs://test-modelarts/code/.
Boot File
Select the Python boot script of the training job in the code directory, for example, obs://test-modelarts/code/main.py.
Hyperparameters
If the resource specification is single-node multi-card, you need to specify the hyperparameters world_size and rank.
If you select a resource flavor with multiple nodes (more than one compute node), you do not need to set these hyperparameters. world_size and rank are automatically injected by ModelArts.
- Method 2: Use a custom image and run the torch.distributed.launch command to start a training job.
For details about parameters for creating a training job, see Table 2.
Table 2 Creating a training job (custom image + torch.distributed.launch) Parameter
Description
Algorithm Type
Select Custom algorithm.
Boot Mode
Select Custom image.
Image
Select a PyTorch image for training.
Code Directory
Select the path where the training code folder is stored in the OBS bucket, for example, obs://test-modelarts/code/.
Boot Command
Enter the Python startup command of the image, for example:
bash ${MA_JOB_DIR}/code/torchlaunch.sh
- Method 3: Use a custom image and run the torch.distributed.run command to start a training job.
For details about parameters for creating a training job, see Table 3.
Table 3 Creating a training job (custom image + torch.distributed.run) Parameter
Description
Algorithm Type
Select Custom algorithm.
Boot Mode
Select Custom image.
Image
Select a PyTorch image for training.
Code Directory
Select the path where the training code folder is stored in the OBS bucket, for example, obs://test-modelarts/code/.
Boot Command
Enter the Python startup command of the image, for example:
bash ${MA_JOB_DIR}/code/torchrun.sh
Code Examples
Upload the following files to an OBS bucket:
code # Root directory of the code └─torch_ddp.py # Code file for PyTorch DDP training └─main.py # Boot file for starting training using the PyTorch preset image and the mp.spawn command └─torchlaunch.sh # Boot file for starting training using the custom image and the torch.distributed.launch command └─torchrun.sh # Boot file for starting training using the custom image and the torch.distributed.run command
torch_ddp.py
import os import torch import torch.distributed as dist import torch.nn as nn import torch.optim as optim from torch.nn.parallel import DistributedDataParallel as DDP # Start training by running mp.spawn. def init_from_arg(local_rank, base_rank, world_size, init_method): rank = base_rank + local_rank dist.init_process_group("nccl", rank=rank, init_method=init_method, world_size=world_size) ddp_train(local_rank) # Start training by running torch.distributed.launch or torch.distributed.run. def init_from_env(): dist.init_process_group(backend='nccl', init_method='env://') local_rank=int(os.environ["LOCAL_RANK"]) ddp_train(local_rank) def cleanup(): dist.destroy_process_group() class ToyModel(nn.Module): def __init__(self): super(ToyModel, self).__init__() self.net1 = nn.Linear(10, 10) self.relu = nn.ReLU() self.net2 = nn.Linear(10, 5) def forward(self, x): return self.net2(self.relu(self.net1(x))) def ddp_train(device_id): # create model and move it to GPU with id rank model = ToyModel().to(device_id) ddp_model = DDP(model, device_ids=[device_id]) loss_fn = nn.MSELoss() optimizer = optim.SGD(ddp_model.parameters(), lr=0.001) optimizer.zero_grad() outputs = ddp_model(torch.randn(20, 10)) labels = torch.randn(20, 5).to(device_id) loss_fn(outputs, labels).backward() optimizer.step() cleanup() if __name__ == "__main__": init_from_env()
main.py
import argparse import torch import torch.multiprocessing as mp parser = argparse.ArgumentParser(description='ddp demo args') parser.add_argument('--world_size', type=int, required=True) parser.add_argument('--rank', type=int, required=True) parser.add_argument('--init_method', type=str, required=True) args, unknown = parser.parse_known_args() if __name__ == "__main__": n_gpus = torch.cuda.device_count() world_size = n_gpus * args.world_size base_rank = n_gpus * args.rank # Call the start function in the DDP sample code. from torch_ddp import init_from_arg mp.spawn(init_from_arg, args=(base_rank, world_size, args.init_method), nprocs=n_gpus, join=True)
#!/bin/bash # Default system environment variables. Do not modify them. MASTER_HOST="$VC_WORKER_HOSTS" MASTER_ADDR="${VC_WORKER_HOSTS%%,*}" MASTER_PORT="6060" JOB_ID="1234" NNODES="$MA_NUM_HOSTS" NODE_RANK="$VC_TASK_INDEX" NGPUS_PER_NODE="$MA_NUM_GPUS" # Custom environment variables to specify the Python script and parameters. PYTHON_SCRIPT=${MA_JOB_DIR}/code/torch_ddp.py PYTHON_ARGS="" CMD="python -m torch.distributed.launch \ --nnodes=$NNODES \ --node_rank=$NODE_RANK \ --nproc_per_node=$NGPUS_PER_NODE \ --master_addr $MASTER_ADDR \ --master_port=$MASTER_PORT \ --use_env \ $PYTHON_SCRIPT \ $PYTHON_ARGS " echo $CMD $CMD
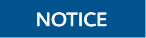
In PyTorch 2.1, you must set rdzv_backend to static: --rdzv_backend=static.
#!/bin/bash # Default system environment variables. Do not modify them. MASTER_HOST="$VC_WORKER_HOSTS" MASTER_ADDR="${VC_WORKER_HOSTS%%,*}" MASTER_PORT="6060" JOB_ID="1234" NNODES="$MA_NUM_HOSTS" NODE_RANK="$VC_TASK_INDEX" NGPUS_PER_NODE="$MA_NUM_GPUS" # Custom environment variables to specify the Python script and parameters. PYTHON_SCRIPT=${MA_JOB_DIR}/code/torch_ddp.py PYTHON_ARGS="" if [[ $NODE_RANK == 0 ]]; then EXT_ARGS="--rdzv_conf=is_host=1" else EXT_ARGS="" fi CMD="python -m torch.distributed.run \ --nnodes=$NNODES \ --node_rank=$NODE_RANK \ $EXT_ARGS \ --nproc_per_node=$NGPUS_PER_NODE \ --rdzv_id=$JOB_ID \ --rdzv_backend=c10d \ --rdzv_endpoint=$MASTER_ADDR:$MASTER_PORT \ $PYTHON_SCRIPT \ $PYTHON_ARGS " echo $CMD $CMD
Feedback
Was this page helpful?
Provide feedbackThank you very much for your feedback. We will continue working to improve the documentation.See the reply and handling status in My Cloud VOC.
For any further questions, feel free to contact us through the chatbot.
Chatbot