Configuring the Software Environment on the GPU Server
Scenario
This section describes how to configure the environment on a GPU BMS, including installing NVIDIA and CUDA drivers. For different GPU preset images, the pre-installed software varies. You can view the installed software by referring to Mapping Between Compute Resources and Image Versions. The following describes the common software installation procedure. You can view the content based on the software to be installed.
- Installing a NVIDIA Driver
- Installing a CUDA Toolkit
- Installing Docker
- Installing nvidia-fabricmanager
The following are the typical configuration scenarios. View the related documents for quick configuration.
Installing a NVIDIA Driver
- Visit the NVIDIA official website.
- The Ant8 specifications are used as an example. Select a driver based on the Ant8 details and the required CUDA version.
Figure 1 Selecting a driverThe driver version is automatically displayed and downloaded.
wget https://cn.download.nvidia.com/tesla/470.182.03/NVIDIA-Linux-x86_64-470.182.03.run
- Assign permissions.
chmod +x NVIDIA-Linux-x86_64-470.182.03.run
- Run the installation file.
./NVIDIA-Linux-x86_64-470.182.03.run
The NVIDIA-DRIVER driver is installed.
Installing a CUDA Toolkit
The NVIDIA driver is installed based on CUDA 12.0. In this case, CUDA 12.0 is installed by default.
- Visit CUDA Toolkit.
- After you set the OS, architecture, distribution, version, and installation type, an installation command is generated. Copy the command and run it.
Figure 2 Settings
The generated installation commands are as follows:
wget https://developer.download.nvidia.com/compute/cuda/repos/ubuntu2004/x86_64/cuda-ubuntu2004.pin sudo mv cuda-ubuntu2004.pin /etc/apt/preferences.d/cuda-repository-pin-600 wget https://developer.download.nvidia.com/compute/cuda/12.1.1/local_installers/cuda-repo-ubuntu2004-12-1-local_12.1.1-530.30.02-1_amd64.deb sudo dpkg -i cuda-repo-ubuntu2004-12-1-local_12.1.1-530.30.02-1_amd64.deb sudo cp /var/cuda-repo-ubuntu2004-12-1-local/cuda-*-keyring.gpg /usr/share/keyrings/ sudo apt-get update sudo apt-get -y install cuda
Installing Docker
Docker is not installed in some preset images of Vnt1 BMS. To install Docker, see the following operations:
- Install Docker.
curl https://get.docker.com | sh && sudo systemctl --now enable docker
- Install the NIVDIA container plug-in.
distribution=$(. /etc/os-release;echo $ID$VERSION_ID) && curl -fsSL https://nvidia.github.io/libnvidia-container/gpgkey | sudo gpg --dearmor -o /usr/share/keyrings/nvidia-container-toolkit-keyring.gpg && curl -s -L https://nvidia.github.io/libnvidia-container/$distribution/libnvidia-container.list | sed 's#deb https://#deb [signed-by=/usr/share/keyrings/nvidia-container-toolkit-keyring.gpg] https://#g' | sudo tee /etc/apt/sources.list.d/nvidia-container-toolkit.list apt-get update apt-get install -y nvidia-container-toolkit nvidia-ctk runtime configure --runtime=docker systemctl restart docker
- Check whether the Docker environment has been installed.
The following uses PyTorch 2.0 as an example. The image used in this case is large and it may take a while to pull the image.
docker run -ti --runtime=nvidia --gpus all pytorch/pytorch:2.0.0-cuda11.7-cudnn8-devel bash
Figure 3 Image pulled
Installing nvidia-fabricmanager
NVLink and NVSwitch are supported for Ant GPUs. If you use a node with multiple GPUs, install nvidia-fabricmanager matching your driver version to enable interconnection between GPUs. Otherwise, GPU pods may fail to be used.
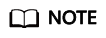
The nvidia-fabricmanager version must be the same as the nvidia driver version.
The following uses version 515.105.01 as an example.
version=515.105.01 main_version=$(echo $version | awk -F '.' '{print $1}') apt-get update apt-get -y install nvidia-fabricmanager-${main_version}=${version}-*
Verify the driver installation result. Start the fabricmanager service and check whether the status is RUNNING.
nvidia-smi -pm 1 nvidia-smi systemctl enable nvidia-fabricmanager systemctl start nvidia-fabricmanager systemctl status nvidia-fabricmanager
Installing NVIDIA 515 and CUDA 11.7 on a GP Vnt1 BMS in EulerOS 2.9
This section describes how to install NVIDIA 515.105.01 and CUDA 11.7.1 on a GP Vnt1 BMS in EulerOS 2.9.
- Install the NVIDIA driver.
wget https://us.download.nvidia.com/tesla/515.105.01/NVIDIA-Linux-x86_64-515.105.01.run chmod 700 NVIDIA-Linux-x86_64-515.105.01.run yum install -y elfutils-libelf-devel ./NVIDIA-Linux-x86_64-515.105.01.run --kernel-source-path=/usr/src/kernels/4.18.0-147.5.1.6.h998.eulerosv2r9.x86_64
By default, the Yum repository used by the Vnt1 BMS is http://repo.huaweicloud.com, which is available. If an error message is displayed when you run the yum update command, indicating that a software package conflict occurs, run the yum remove xxx software package command.
The NVIDIA driver is a binary file and requires the libelf library in the elfutils-libelf-devel development package in the system. It provides a set of C functions for reading, modifying, and creating ELF files. NVIDIA drivers need these functions to parse the currently running kernel and other related information.
During the installation, select OK or YES as prompted. After the installation, run the reboot command to restart the server. Log in to the server again and run the following command to view the GPU information:
nvidia-smi -pm 1 #This command will be executed for a while. Wait patiently. The persistent mode is enabled to optimize the GPU performance on the Linux instance. nvidia-smi
- Install CUDA.
wget https://developer.download.nvidia.com/compute/cuda/11.7.1/local_installers/cuda_11.7.1_515.65.01_linux.run chmod 700 cuda_11.7.1_515.65.01_linux.run ./cuda_11.7.1_515.65.01_linux.run --toolkit --samples --silent
Check the installation result.
/usr/local/cuda/bin/nvcc -V
- Install PyTorch 2.0 and verify CUDA.
To install PyTorch 2.0, Python 3.10 is required, and the miniconda environment needs to be installed and configured.
- Install miniconda and create the alpha environment.
wget https://repo.anaconda.com/miniconda/Miniconda3-py310_23.1.0-1-Linux-x86_64.sh chmod 750 Miniconda3-py310_23.1.0-1-Linux-x86_64.sh bash Miniconda3-py310_23.1.0-1-Linux-x86_64.sh -b -p /home/miniconda export PATH=/home/miniconda/bin:$PATH conda create --quiet --yes -n alpha python=3.10
- Install PyTorch 2.0 and verify the CUDA status.
Install PyTorch 2.0 in the alpha environment and use the Tsinghua PIP source.
source activate alpha pip install torch==2.0 -i https://pypi.tuna.tsinghua.edu.cn/simple python
Verify the installation status of PyTorch and CUDA. If the output is True, the installation is successful.import torch print(torch.cuda.is_available())
- Install miniconda and create the alpha environment.
Installing NVIDIA 470 and CUDA 11.4 on a GP Vnt1 BMS in Ubuntu 18.04
This section describes how to install NVIDIA 470 and CUDA 11.4 on a GP Vnt1 BMS in Ubuntu 18.04.
- Install the NVIDIA driver.
apt-get update sudo apt-get install nvidia-driver-470
- Install CUDA.
wget https://developer.download.nvidia.com/compute/cuda/11.4.4/local_installers/cuda_11.4.4_470.82.01_linux.run chmod +x cuda_11.4.4_470.82.01_linux.run ./cuda_11.4.4_470.82.01_linux.run --toolkit --samples --silent
- Verify the NVIDIA installation result.
nvidia-smi -pm 1 nvidia-smi /usr/local/cuda/bin/nvcc -V
- Install PyTorch 2.0 and verify CUDA.
To install PyTorch 2.0, Python 3.10 is required, and the miniconda environment needs to be installed and configured.
- Install miniconda and create the alpha environment.
wget https://repo.anaconda.com/miniconda/Miniconda3-py310_23.1.0-1-Linux-x86_64.sh chmod 750 Miniconda3-py310_23.1.0-1-Linux-x86_64.sh bash Miniconda3-py310_23.1.0-1-Linux-x86_64.sh -b -p /home/miniconda export PATH=/home/miniconda/bin:$PATH conda create --quiet --yes -n alpha python=3.10
- Install PyTorch 2.0 and verify the CUDA status.
Install PyTorch 2.0 in the alpha environment and use the Tsinghua PIP source.
source activate alpha conda install pytorch torchvision torchaudio pytorch-cuda=11.7 -c pytorch -c nvidia python
Verify the installation status of PyTorch and CUDA. If the output is True, the installation is successful.import torch print(torch.cuda.is_available())
- Install miniconda and create the alpha environment.
Installing NVIDIA 515 and CUDA 11.7 on a GP Vnt1 BMS in Ubuntu18.04
This section describes how to install NVIDIA 515 and CUDA 11.7 on a GP Vnt1 BMS in Ubuntu18.04.
- Install the NVIDIA driver.
wget https://us.download.nvidia.com/tesla/515.105.01/NVIDIA-Linux-x86_64-515.105.01.run chmod +x NVIDIA-Linux-x86_64-515.105.01.run ./NVIDIA-Linux-x86_64-515.105.01.run
- Install CUDA.
wget https://developer.download.nvidia.com/compute/cuda/11.7.1/local_installers/cuda_11.7.1_515.65.01_linux.run chmod +x cuda_11.7.1_515.65.01_linux.run ./cuda_11.7.1_515.65.01_linux.run --toolkit --samples –silent
- Install Docker.
curl https://get.docker.com | sh && sudo systemctl --now enable docker
- Install the NIVDIA container plug-in.
distribution=$(. /etc/os-release;echo $ID$VERSION_ID) && curl -fsSL https://nvidia.github.io/libnvidia-container/gpgkey | sudo gpg --dearmor -o /usr/share/keyrings/nvidia-container-toolkit-keyring.gpg && curl -s -L https://nvidia.github.io/libnvidia-container/$distribution/libnvidia-container.list | sed 's#deb https://#deb [signed-by=/usr/share/keyrings/nvidia-container-toolkit-keyring.gpg] https://#g' | sudo tee /etc/apt/sources.list.d/nvidia-container-toolkit.list apt-get update apt-get install -y nvidia-container-toolkit nvidia-ctk runtime configure --runtime=docker systemctl restart docker
- Check whether the Docker environment has been installed.
The following uses PyTorch 2.0 as an example. The image used in this case is large and it may take a while to pull the image.
docker run -ti --runtime=nvidia --gpus all pytorch/pytorch:2.0.0-cuda11.7-cudnn8-devel bash
Figure 4 Image pulled
Installing NVIDIA 515 and CUDA 11.7 on a GP Ant8 BMS in Ubuntu 20.04
This section describes how to install NVIDIA driver 515, CUDA 11.7, and nvidia-fabricmanager 515 on GP Ant8 BMS (Ubuntu 20.04) and perform the nccl-test test.
- Replace the APT source.
sudo sed -i "s@http://.*archive.ubuntu.com@http://repo.huaweicloud.com@g" /etc/apt/sources.list sudo sed -i "s@http://.*security.ubuntu.com@http://repo.huaweicloud.com@g" /etc/apt/sources.list sudo apt update
- Install the NVIDIA driver.
wget https://us.download.nvidia.com/tesla/515.105.01/NVIDIA-Linux-x86_64-515.105.01.run chmod +x NVIDIA-Linux-x86_64-515.105.01.run ./NVIDIA-Linux-x86_64-515.105.01.run
- Install CUDA.
# Install the .run package. wget https://developer.download.nvidia.com/compute/cuda/11.7.0/local_installers/cuda_11.7.0_515.43.04_linux.run chmod +x cuda_11.7.0_515.43.04_linux.run ./cuda_11.7.0_515.43.04_linux.run --toolkit --samples --silent
- Install NCCL.
- For details about how to install NCCL, see NVIDIA Deep Learning NCCL Documentation.
- For details about the mapping between NCCL and CUDA versions and the installation method, see NCL Developer.
The following uses CUDA 11.7 as an example. Install NCCL.
wget https://developer.download.nvidia.com/compute/cuda/repos/ubuntu2004/x86_64/cuda-keyring_1.0-1_all.deb sudo dpkg -i cuda-keyring_1.0-1_all.deb sudo apt update sudo apt install libnccl2=2.14.3-1+cuda11.7 libnccl-dev=2.14.3-1+cuda11.7
The following is displayed after NCCL is installed.
Figure 5 Viewing NCCL - Install nvidia-fabricmanager.
The nvidia-fabricmanager version must be the same as the nvidia driver version.
version=515.105.01 main_version=$(echo $version | awk -F '.' '{print $1}') apt-get update apt-get -y install nvidia-fabricmanager-${main_version}=${version}-*
Verify the driver installation result. Start the fabricmanager service and check whether the status is RUNNING.
nvidia-smi -pm 1 nvidia-smi systemctl enable nvidia-fabricmanager systemctl start nvidia-fabricmanager systemctl status nvidia-fabricmanager
- Install nv-peer-memory.
git clone https://github.com/Mellanox/nv_peer_memory.git cd ./nv_peer_memory ./build_module.sh cd /tmp tar xzf /tmp/nvidia-peer-memory_1.3.orig.tar.gz cd nvidia-peer-memory-1.3 dpkg-buildpackage -us -uc dpkg -i ../nvidia-peer-memory-dkms_1.2-0_all.deb
nv_peer_mem works in Linux kernel mode. Run the lsmod | grep peer command to check whether nv_peer_mem is loaded to the kernel.
- If the code cannot be pulled by running the git clone command, configure git.
git config --global core.compression -1 export GIT_SSL_NO_VERIFY=1 git config --global http.sslVerify false git config --global http.postBuffer 10524288000 git config --global http.lowSpeedLimit 1000 git config --global http.lowSpeedTime 1800
- If nv-peer-memory is not displayed after the installation, the InfiniBand driver version may be too early. In this case, upgrade InfiniBand.
wget https://content.mellanox.com/ofed/MLNX_OFED-5.4-3.6.8.1/MLNX_OFED_LINUX-5.4-3.6.8.1-ubuntu20.04-x86_64.tgz tar -zxvf MLNX_OFED_LINUX-5.4-3.6.8.1-ubuntu20.04-x86_64.tgz cd MLNX_OFED_LINUX-5.4-3.6.8.1-ubuntu20.04-x86_64 apt-get install -y python3 gcc quilt build-essential bzip2 dh-python pkg-config dh-autoreconf python3-distutils debhelper make ./mlnxofedinstall --add-kernel-support
- For details about how to install a later version, see Linux InfiniBand Drivers. For example, install the latest version, which is MLNX_OFED-5.8-2.0.3.0.
wget https://content.mellanox.com/ofed/MLNX_OFED-5.8-2.0.3.0/MLNX_OFED_LINUX-5.8-2.0.3.0-ubuntu20.04-x86_64.tgz tar -zxvf MLNX_OFED_LINUX-5.8-2.0.3.0-ubuntu20.04-x86_64.tgz cd MLNX_OFED_LINUX-5.8-2.0.3.0-ubuntu20.04-x86_64 apt-get install -y python3 gcc quilt build-essential bzip2 dh-python pkg-config dh-autoreconf python3-distutils debhelper make ./mlnxofedinstall --add-kernel-support
- After nv_peer_mem is installed, view its status.
/etc/init.d/nv_peer_mem/ status
If the file does not exist, the file may not be copied by default during the installation. In this case, you need to copy the file.
cp /tmp/nvidia-peer-memory-1.3/nv_peer_mem.conf /etc/infiniband/ cp /tmp/nvidia-peer-memory-1.3/debian/tmp/etc/init.d/nv_peer_mem /etc/init.d/
- If the code cannot be pulled by running the git clone command, configure git.
- Configure environment variables.
The MPI path version must match. You can run the ls /usr/mpi/gcc/ command to view the Open MPI version.
# Add to ~/.bashrc. export LD_LIBRARY_PATH=/usr/local/cuda/lib:usr/local/cuda/lib64:/usr/include/nccl.h:/usr/mpi/gcc/openmpi-4.1.2a1/lib:$LD_LIBRARY_PATH export PATH=$PATH:/usr/local/cuda/bin:/usr/mpi/gcc/openmpi-4.1.2a1/bin
- Install and compile nccl-test.
cd /root git clone https://github.com/NVIDIA/nccl-tests.git cd ./nccl-tests make MPI=1 MPI_HOME=/usr/mpi/gcc/openmpi-4.1.2a1 -j 8
The parameter MPI=1 must be added during compilation. Otherwise, the test between multiple devices cannot be performed.
The MPI path version must match. You can run the ls /usr/mpi/gcc/ command to view the Open MPI version.
- Perform the nccl-test test.
- Single-server test:
/root/nccl-tests/build/all_reduce_perf -b 8 -e 1024M -f 2 -g 8
- Multi-server test (replace the content comes after btl_tcp_if_include with the active NIC name):
mpirun --allow-run-as-root --hostfile hostfile -mca btl_tcp_if_include eth0 -mca btl_openib_allow_ib true -x NCCL_DEBUG=INFO -x NCCL_IB_GID_INDEX=3 -x NCCL_IB_TC=128 -x NCCL_ALGO=RING -x NCCL_IB_HCA=^mlx5_bond_0 -x LD_LIBRARY_PATH /root/nccl-tests/build/all_reduce_perf -b 8 -e 11g -f 2 -g 8
hostfile format:
#Private IP address of the host Number of processes on a single node 192.168.20.1 slots=1 192.168.20.2 slots=1
NCCL environment variables:
- NCCL_IB_GID_INDEX=3: enables data packets to be transmitted through the queue 4 of switches, which is RoCE-compliant.
- NCCL_IB_TC=128: enables RoCEv2. RoCEv1 is enabled by default. However, RoCEv1 does not support congestion control on switches, which may lead to packet loss. In addition, later-version switches do not support RoCEv1, leading to a RoCEv1 failure.
- NCCL_ALGO=RING: The bus bandwidth of nccl_test is calculated based on the ring algorithm.
The calculation formulas are as follows: Bus bandwidth = Algorithm bandwidth x 2(N-1)/N, Algorithm bandwidth = Data volume/Time
The ring algorithm must be used. The formulas are different for the tree algorithm.
The bus bandwidth calculated by the tree algorithm is equivalent to the performance acceleration compared with the ring algorithm. The total time required for algorithm calculation is reduced. Therefore, the bus bandwidth calculated using the formula is also increased. Theoretically, the tree algorithm is better than the ring algorithm. However, the tree algorithm has higher requirements on the network than the ring algorithm, and the calculation may be unstable. The tree algorithm can complete the all reduce calculation with less data traffic, but it is not suitable for testing performance. Therefore, the actual bandwidth of two nodes is 100 GB/s, but the tested speed is 110 GB/s or even 130 GB/s. After this parameter is added, the speed is stable in the case of two or more nodes.
During the test, password-free login is required between the node where the mpirun command is executed and the node in the hostfile. To set SSH password-free login, perform the following steps:- Generate a pair of public and private keys on the local client.
ssh-keygen
After the preceding command is executed, id_rsa.pub (public key) and id_rsa (private key) are created in the .ssh folder in the user directory. View the public key and private key.
cd ~/.ssh
- Upload the public key to the server.
For example, if the username is root and the server address is 192.168.222.213.
ssh-copy-id -i ~/.ssh/id_rsa.pub root@192.168.222.213
View the id_rsa.pub (public key) content.
cd ~/.ssh vim authorized_keys
- Test password-free login.
The client connects to the remote server through SSH. You can log in to the server without entering a password.
ssh root@192.168.222.213
- Generate a pair of public and private keys on the local client.
- Single-server test:
Feedback
Was this page helpful?
Provide feedbackThank you very much for your feedback. We will continue working to improve the documentation.See the reply and handling status in My Cloud VOC.
For any further questions, feel free to contact us through the chatbot.
Chatbot