Creating a Single-Node Multi-Card Distributed Training Job (DataParallel)
This section describes how to perform single-node multi-card parallel training based on the PyTorch engine.
For details about the distributed training using the MindSpore engine, see the MindSpore official website.
Training Process
The process of single-node multi-card parallel training is as follows:
- A model is copied to multiple GPUs.
- Data of each batch is distributed evenly to each worker GPU.
- Each GPU does its own forward propagation and an output is obtained.
- The master GPU with device ID 0 collects the output of each GPU and calculates the loss.
- The master GPU distributes the loss to each worker GPU. Each GPU does its own backward propagation and calculates the gradient.
- The master GPU collects gradients, updates parameter settings, and distributes the settings to each worker GPU.
The detailed flowchart is as follows.
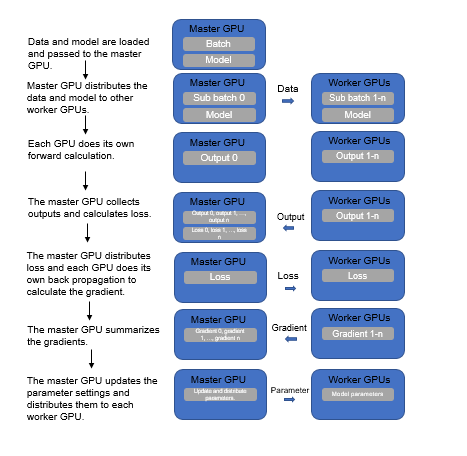
Code Modifications
Model distribution: DataParallel(model)
The code is slightly changed and the following is a simple example:
import torch class Net(torch.nn.Module): pass model = Net().cuda() ### DataParallel Begin ### model = torch.nn.DataParallel(Net().cuda()) ### DataParallel End ###
Feedback
Was this page helpful?
Provide feedbackThank you very much for your feedback. We will continue working to improve the documentation.See the reply and handling status in My Cloud VOC.
For any further questions, feel free to contact us through the chatbot.
Chatbot