Model Training Process
AI modeling involves two stages:
- Development: Prepare and configure the environment, and debug code for training based on deep learning. ModelArts DevEnviron is recommended for code debugging.
- Experiment: Optimize the datasets and hyperparameters, and obtain an ideal model through multiple rounds of experiments. The ModelArts training platform is recommended for training.
In the two stages, code is designed, developed and tested in repeated cycles. In the development stage, when the code becomes stable, the modeling process enters the experiment stage, during which hyperparameters are continuously optimized to iterate the model. In the experiment stage, when the training performance can be optimized, the modeling process returns to the development stage for optimizing code.
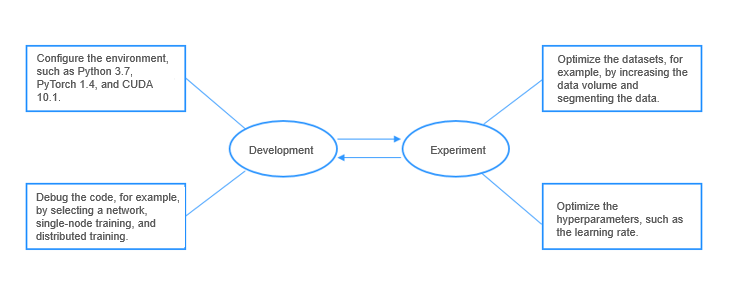
ModelArts provides model training, which allows you to view training results and tune model parameters based on the training results. You can select resource pools with different instance flavors for model training.
To train a model on ModelArts Standard, follow these steps:
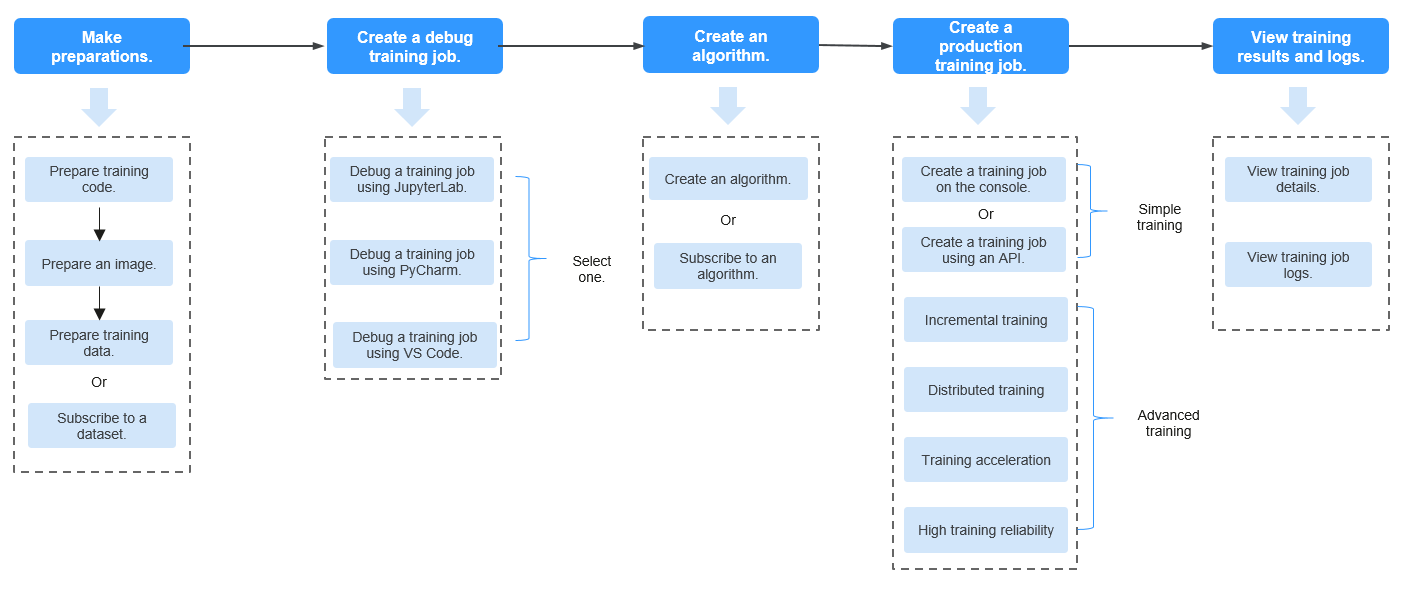
Task |
Subtask |
Description |
---|---|---|
Making preparations |
Preparing training code |
Model training includes training code, training framework, and training data.
Training code contains the boot file or command and dependency package of a training job.
|
Preparing a training image |
There are multiple training image sources. For details, see Preparing a Model Training Image.
|
|
Preparing training data |
Before training, prepare necessary data, which can be datasets or predictive models.
|
|
Creating a debug training job |
Creating a debug training job |
Before model training, debug your code. ModelArts offers multiple methods for creating a debug training job.
|
Creating an algorithm |
Creating an algorithm |
Before creating a production training job, create an algorithm or subscribe to an algorithm from AI Gallery. |
Creating a production training job |
Using basic training features |
|
Using advanced training features |
ModelArts Standard supports the following advanced training features:
|
|
Viewing training results and logs |
Viewing training job details |
You can view a training job's parameter settings and events on the job details page at any time, whether the job is running or has completed. |
Viewing training job logs |
Training logs track the execution and any errors that occur during training job runs. You can view these logs to identify and troubleshoot issues that cause jobs to fail. |
Creation Method |
Description |
---|---|
Using a preset image to create a training job |
If you have used some mainstream images to develop algorithms locally, you can select a mainstream image and create a training job to build a model. |
Using a custom image to create a training job |
To use a non-mainstream image, create a custom algorithm image and then use it to create a training job. |
Using an existing algorithm to create a training job |
In Algorithm Management, you can manage your created algorithms and those subscribed from AI Gallery. This allows you to quickly create training jobs and build models using these algorithms. |
Using a subscribed algorithm to create a training job |
You can subscribe to algorithms in AI Gallery to quickly create training jobs and build models. |
Feedback
Was this page helpful?
Provide feedbackThank you very much for your feedback. We will continue working to improve the documentation.See the reply and handling status in My Cloud VOC.
For any further questions, feel free to contact us through the chatbot.
Chatbot