查看模型评估结果
训练作业运行结束后,ModelArts可为您的模型进行评估,并且给出调优诊断和建议。
- 针对使用预置算法创建训练作业,无需任何配置,即可查看此评估结果(由于每个模型情况不同,系统将自动根据您的模型指标情况,给出一些调优建议,请仔细阅读界面中的建议和指导,对您的模型进行进一步的调优)。
- 针对用户自己编写训练脚本或自定义镜像方式创建的训练作业,则需要在您的训练代码中添加评估代码,才可以在训练作业结束后查看相应的评估诊断建议。
- 只支持验证集的数据格式为图片。
- 目前,仅如下常用框架的训练脚本支持添加评估代码。
- TF-1.13.1-python3.6
- TF-2.1.0-python3.6
- PyTorch-1.4.0-python3.6
下文将介绍如何在训练中使用评估代码。对训练代码做一定的适配和修正,分为三个方面:添加输出目录、复制数据集到本地、映射数据集路径到OBS。
添加输出目录
添加输出目录的代码比较简单,即在代码中添加一个输出评估结果文件的目录,被称为train_url,也就是页面上的训练输出位置。并把train_url添加到使用的函数analysis中,使用save_path来获取train_url。示例代码如下所示:
1 2 3 4 5 6 7 8 9 10 11 12 13 14 15 16 17 18 19 |
FLAGS = tf.app.flags.FLAGS tf.app.flags.DEFINE_string('model_url', '', 'path to saved model') tf.app.flags.DEFINE_string('data_url', '', 'path to output files') tf.app.flags.DEFINE_string('train_url', '', 'path to output files') tf.app.flags.DEFINE_string('adv_param_json', '{"attack_method":"FGSM","eps":40}', 'params for adversarial attacks') FLAGS(sys.argv, known_only=True) ... # analyse res = analyse( task_type=task_type, pred_list=pred_list, label_list=label_list, name_list=file_name_list, label_map_dict=label_dict, save_path=FLAGS.train_url) |
复制数据集到本地
复制数据集到本地主要是为了防止长时间访问OBS容易导致OBS连接中断使得作业卡住,所以一般先将数据复制到本地再进行操作。
数据集复制有两种方式,推荐使用OBS路径复制。
- OBS路径(推荐)
- ModelArts数据管理中的数据集(即manifest文件格式)
使用moxing的copy_manifest接口将文件复制到本地并获取新的manifest文件路径,然后使用SDK解析新的manifest文件。
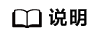
ModelArts数据管理模块在重构升级中,对未使用过数据管理的用户不可见。建议新用户将训练数据存放至OBS桶中使用。
1 2 3 4 5 6 7 8 |
if data_path.startswith('obs://'): if '.manifest' in data_path: new_manifest_path, _ = mox.file.copy_manifest(data_path, '/cache/data/') data_path = new_manifest_path else: mox.file.copy_parallel(data_path, '/cache/data/') data_path = '/cache/data/' print('------------- download dataset success ------------') |
映射数据集路径到OBS
由于最终JSON体中需要填写的是图片文件的真实路径,也就是OBS对应的路径,所以在复制到本地做完分析和评估操作后,需要将原来的本地数据集路径映射到OBS路径,然后将新的list送入analysis接口。
如果使用的是OBS路径作为输入的data_url,则只需要替换本地路径的字符串即可。
1 2 3 |
if FLAGS.data_url.startswith('obs://'): for idx, item in enumerate(file_name_list): file_name_list[idx] = item.replace(data_path, FLAGS.data_url) |
如果使用manifest文件,需要再解析一遍原版的manifest文件获取list,然后再送入analysis接口。
1 2 3 4 5 6 7 8 |
if or FLAGS.data_url.startswith('obs://'): if 'manifest' in FLAGS.data_url: file_name_list = [] manifest, _ = get_sample_list( manifest_path=FLAGS.data_url, task_type='image_classification') for item in manifest: if len(item[1]) != 0: file_name_list.append(item[0]) |
完整的适配了训练作业创建的图像分类样例代码如下:
1 2 3 4 5 6 7 8 9 10 11 12 13 14 15 16 17 18 19 20 21 22 23 24 25 26 27 28 29 30 31 32 33 34 35 36 37 38 39 40 41 42 43 44 45 46 47 48 49 50 51 52 53 54 55 56 57 58 59 60 61 62 63 64 65 66 67 68 69 70 71 72 73 74 75 76 77 78 79 80 81 82 83 84 85 86 87 88 89 90 91 92 93 94 95 96 97 98 99 100 101 102 103 104 105 106 107 108 109 110 111 112 113 114 115 116 117 118 119 120 121 122 123 124 125 126 127 128 129 130 131 132 133 134 135 136 137 138 139 140 141 142 143 144 145 146 147 148 149 150 151 152 153 154 155 156 157 158 159 160 161 162 163 164 165 166 167 168 169 170 171 172 173 174 175 176 177 178 179 180 |
import json import logging import os import sys import tempfile import h5py import numpy as np from PIL import Image import moxing as mox import tensorflow as tf from deep_moxing.framework.manifest_api.manifest_api import get_sample_list from deep_moxing.model_analysis.api import analyse, tmp_save from deep_moxing.model_analysis.common.constant import TMP_FILE_NAME logging.basicConfig(level=logging.DEBUG) FLAGS = tf.app.flags.FLAGS tf.app.flags.DEFINE_string('model_url', '', 'path to saved model') tf.app.flags.DEFINE_string('data_url', '', 'path to output files') tf.app.flags.DEFINE_string('train_url', '', 'path to output files') tf.app.flags.DEFINE_string('adv_param_json', '{"attack_method":"FGSM","eps":40}', 'params for adversarial attacks') FLAGS(sys.argv, known_only=True) def _preprocess(data_path): img = Image.open(data_path) img = img.convert('RGB') img = np.asarray(img, dtype=np.float32) img = img[np.newaxis, :, :, :] return img def softmax(x): x = np.array(x) orig_shape = x.shape if len(x.shape) > 1: # Matrix x = np.apply_along_axis(lambda x: np.exp(x - np.max(x)), 1, x) denominator = np.apply_along_axis(lambda x: 1.0 / np.sum(x), 1, x) if len(denominator.shape) == 1: denominator = denominator.reshape((denominator.shape[0], 1)) x = x * denominator else: # Vector x_max = np.max(x) x = x - x_max numerator = np.exp(x) denominator = 1.0 / np.sum(numerator) x = numerator.dot(denominator) assert x.shape == orig_shape return x def get_dataset(data_path, label_map_dict): label_list = [] img_name_list = [] if 'manifest' in data_path: manifest, _ = get_sample_list( manifest_path=data_path, task_type='image_classification') for item in manifest: if len(item[1]) != 0: label_list.append(label_map_dict.get(item[1][0])) img_name_list.append(item[0]) else: continue else: label_name_list = os.listdir(data_path) label_dict = {} for idx, item in enumerate(label_name_list): label_dict[str(idx)] = item sub_img_list = os.listdir(os.path.join(data_path, item)) img_name_list += [ os.path.join(data_path, item, img_name) for img_name in sub_img_list ] label_list += [label_map_dict.get(item)] * len(sub_img_list) return img_name_list, label_list def deal_ckpt_and_data_with_obs(): pb_dir = FLAGS.model_url data_path = FLAGS.data_url if pb_dir.startswith('obs://'): mox.file.copy_parallel(pb_dir, '/cache/ckpt/') pb_dir = '/cache/ckpt' print('------------- download success ------------') if data_path.startswith('obs://'): if '.manifest' in data_path: new_manifest_path, _ = mox.file.copy_manifest(data_path, '/cache/data/') data_path = new_manifest_path else: mox.file.copy_parallel(data_path, '/cache/data/') data_path = '/cache/data/' print('------------- download dataset success ------------') assert os.path.isdir(pb_dir), 'Error, pb_dir must be a directory' return pb_dir, data_path def evalution(): pb_dir, data_path = deal_ckpt_and_data_with_obs() index_file = os.path.join(pb_dir, 'index') try: label_file = h5py.File(index_file, 'r') label_array = label_file['labels_list'][:].tolist() label_array = [item.decode('utf-8') for item in label_array] except Exception as e: logging.warning(e) logging.warning('index file is not a h5 file, try json.') with open(index_file, 'r') as load_f: label_file = json.load(load_f) label_array = label_file['labels_list'][:] label_map_dict = {} label_dict = {} for idx, item in enumerate(label_array): label_map_dict[item] = idx label_dict[idx] = item print(label_map_dict) print(label_dict) data_file_list, label_list = get_dataset(data_path, label_map_dict) assert len(label_list) > 0, 'missing valid data' assert None not in label_list, 'dataset and model not match' pred_list = [] file_name_list = [] img_list = [] for img_path in data_file_list: img = _preprocess(img_path) img_list.append(img) file_name_list.append(img_path) config = tf.ConfigProto() config.gpu_options.allow_growth = True config.gpu_options.visible_device_list = '0' with tf.Session(graph=tf.Graph(), config=config) as sess: meta_graph_def = tf.saved_model.loader.load( sess, [tf.saved_model.tag_constants.SERVING], pb_dir) signature = meta_graph_def.signature_def signature_key = 'predict_object' input_key = 'images' output_key = 'logits' x_tensor_name = signature[signature_key].inputs[input_key].name y_tensor_name = signature[signature_key].outputs[output_key].name x = sess.graph.get_tensor_by_name(x_tensor_name) y = sess.graph.get_tensor_by_name(y_tensor_name) for img in img_list: pred_output = sess.run([y], {x: img}) pred_output = softmax(pred_output[0]) pred_list.append(pred_output[0].tolist()) label_dict = json.dumps(label_dict) task_type = 'image_classification' if FLAGS.data_url.startswith('obs://'): if 'manifest' in FLAGS.data_url: file_name_list = [] manifest, _ = get_sample_list( manifest_path=FLAGS.data_url, task_type='image_classification') for item in manifest: if len(item[1]) != 0: file_name_list.append(item[0]) for idx, item in enumerate(file_name_list): file_name_list[idx] = item.replace(data_path, FLAGS.data_url) # analyse res = analyse( task_type=task_type, pred_list=pred_list, label_list=label_list, name_list=file_name_list, label_map_dict=label_dict, save_path=FLAGS.train_url) if __name__ == "__main__": evalution() |