部署本地服务进行调试
可以通过部署本地服务来进行调试,即在导入模型或模型调试后,在本地部署Predictor进行本地推理,不再依赖线上资源。
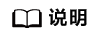
目前仅支持在Linux平台上部署本地服务Predictor,Windows平台不支持。推荐使用ModelArts Notebook部署本地服务。
- 本地服务Predictor和在线服务Predictor说明
- 部署本地服务Predictor,即将模型文件部署在本地,其环境规格取决于本地;例如在一个modelarts.vm.cpu.2u的Notebook中,部署本地Predictor,其运行环境就是cpu.2u。
- 部署在线服务Predictor,即将存储在OBS中的模型文件部署到线上服务管理模块提供的容器中运行,其环境规格(如CPU规格,GPU规格)由表3 predictor configs结构决定。
- 部署在线服务Predictor需要线上服务端根据AI引擎创建容器,较耗时;本地Predictor部署较快,最长耗时10s,可用以测试模型,不建议进行模型的工业应用。
- 当前版本支持部署本地服务Predictor的AI引擎为:“XGBoost”、“Scikit_Learn”、“PyTorch”、“TensorFlow”和“Spark_MLlib”。具体版本信息可参考支持的常用引擎及其Runtime。
示例代码
在ModelArts notebook平台,Session鉴权无需输入鉴权参数。其它平台的Session鉴权请参见Session鉴权。
TensorFlow1.8本地推理示例代码
需要在环境中配置“tensorflow_model_server”,可调用SDK接口快速配置,请参考如下示例代码。
- CPU环境,调用Model.configure_tf_infer_environ(device_type="CPU")完成配置,环境中只需配置运行一次。
- GPU环境,调用Model.configure_tf_infer_environ(device_type="GPU")完成配置,环境中只需配置运行一次。
1 2 3 4 5 6 7 8 9 10 11 12 13 14 15 16 17 18 19 20 21 22 23 24 25 26 27 28 29 |
from modelarts.session import Session from modelarts.model import Model from modelarts.config.model_config import ServiceConfig session = Session() # GPU环境推理配置 Model.configure_tf_infer_environ(device_type="GPU") # CPU环境推理配置 #Model.configure_tf_infer_environ(device_type="CPU") model_instance = Model( session, model_name="input_model_name", # 模型名称 model_version="1.0.0", # 模型版本 source_location=model_location, # 模型文件路径 model_type="MXNet", # 模型类型 model_algorithm="image_classification", # 模型算法 execution_code="OBS_PATH", input_params=input_params, # 参考input_params格式描述 output_params=output_params, # 参考output_params格式描述 dependencies=dependencies, # 参考dependencies格式描述 apis=apis) configs = [ServiceConfig(model_id=model_instance.get_model_id(), weight="100", instance_count=1, specification="local")] predictor_instance = model_instance.deploy_predictor(configs=configs) if predictor_instance is not None: predict_result = predictor_instance.predict(data="your_raw_data_or_data_path", data_type="your_data_type") # 本地推理预测,data支持raw data或者文件路径,data_type支持'json'、'files'或者'images' print(predict_result) |
参数说明
参数 |
是否必选 |
参数类型 |
描述 |
---|---|---|---|
service_name |
否 |
String |
服务名称,支持1-64位可见字符(含中文),只能以英文大小写字母或者中文字符开头,名称可以包含字母、中文、数字、中划线、下划线。 |
configs |
是 |
JSON Array |
本地服务运行配置。 |
参数 |
是否必选 |
参数类型 |
描述 |
---|---|---|---|
model_id |
是 |
String |
模型ID。“model_id”可以通过查询模型列表或者ModelArts管理控制台获取。 |
weight |
是 |
Integer |
权重百分比,分配到此模型的流量权重,部署本地服务Predictor时,取值100。 |
specification |
是 |
String |
部署本地服务时,取值为“local”。 |
instance_count |
是 |
Integer |
模型部署的实例数,当前限制最大实例数为128,部署本地服务Predictor时,取值为1。 |
envs |
否 |
Map<String, String> |
运行模型需要的环境变量键值对,可选填,默认为空。 |
参数 |
是否必选 |
参数类型 |
描述 |
---|---|---|---|
predictor |
是 |
Predictor对象 |
Predictor对象,其属性只包括推理服务测试。 |