聚合函数
聚合函数对一组值进行运算,最终获得一个单值。
除count()、count_if()、max_by()、min_by()和approx_distinct()外,其它聚合函数都忽略空值,并在没有输入行或所有值都为空时返回空值。例如sum()返回null而不是零,并且avg()在统计时不会包含null值。coalesce函数可用于将null转换为零。
聚合函数的子句
- 排序order by
有些聚合函数可能会因为输入值的顺序不同而导致产生不同的结果,可以通过在聚合函数中使用order by子句来指定此顺序。
array_agg(x ORDER BY y DESC); array_agg(x ORDER BYx,y,z);
- 过滤filter
使用filter关键字可以在聚合的过程中,通过使用where的条件表达式来过滤掉不需要的行。所有的聚合函数都支持这个功能。
aggregate_function(...) FILTER (WHERE <condition>)
示例:
--建表 create table fruit (name varchar, price int); --插入数据 insert into fruit values ('peach',5),('apple',2); --排序 select array_agg (name order by price) from fruit;-- [apple, peach] --过滤 select array_agg(name) filter (where price<10) from fruit;-- [peach, apple]
常用聚合函数
- arbitrary(x)
select arbitrary(price) from fruit;-- 5
- array_agg(x)
描述:返回由输入的x字段构成的数组,元素类型和输入字段一样。
select array_agg(price) from fruit;-- [5,2]
- avg(x)
select avg(price) from fruit;-- 3.5
- avg(time interval type)
描述: 返回所有输入时间间隔的平均长度,返回类型为 interval。
select avg(last_login) from (values ('admin',interval '0 06:15:30' day to second),('user1',interval '0 07:15:30' day to second),('user2',interval '0 08:15:30' day to second)) as login_log(user,last_login); -- 0 07:15:30.000 假设有日志表记录用户距离上次登录的时间,那么这个结果表明平均登录时间间隔为0天7小时15分钟30秒
- bool_and(boolean value)
描述:当每个输入值都是true,返回true,否则返回false。
select bool_and(isfruit) from (values ('item1',true), ('item2',false),('item3',true)) as items(item,isfruit);--false select bool_and(isfruit) from (values ('item1',true), ('item2',true),('item3',true)) as items(item,isfruit);-- true
- bool_or(boolean value)
描述:只要输入值中有为true的,返回true,否则返回false。
select bool_or(isfruit) from (values ('item1',false), ('item2',false),('item3',false)) as items(item,isfruit);-- false select bool_or(isfruit) from (values ('item1',true), ('item2',false),('item3',false)) as items(item,isfruit); --true
- checksum(x)
描述:返回输入值的检查和,其值不受输入顺序影响,结果类型为varbinary。
select checksum(price) from fruit; -- fb 28 f3 9a 9a fb bf 86
- count(*)
select count(*) from fruit; -- 2
- count(x)
描述:返回输入字段非null值的记录条数,结果类型为bigint。
select count(name) from fruit;-- 2
- count_if(x)
描述:类似于count(CASE WHEN x THEN 1 END),返回输入值为true的记录数,bigint类型。
select count_if(price>7) from fruit;-- 0
- every(boolean)
- geometric_mean(x)
select geometric_mean(price) from fruit; -- 3.162277660168379
- listagg(x, separator) → varchar
语法:
LISTAGG( expression [, separator] [ON OVERFLOW overflow_behaviour]) WITHIN GROUP (ORDER BY sort_item, [...])
如果separator未指定, 将默认使用空字符作为分隔符。
SELECT listagg(value, ',') WITHIN GROUP (ORDER BY value) csv_value FROM (VALUES 'a', 'c', 'b') t(value); csv_value ----------- 'a,b,c'
当该函数的输出值超过了1048576字节时,overflow_behaviour 可以指定这种情况下的行为,默认是抛出一个Error:
SELECT listagg(value, ',' ON OVERFLOW ERROR) WITHIN GROUP (ORDER BY value) csv_value FROM (VALUES 'a', 'b', 'c') t(value);
也可以是当函数输出长度超出1048576字节,截断超出非空字符串,并用TRUNCATE 指定的字符串替代,WITH COUNT和WITHOUT COUNT,表示输出结果是否包含计数:
SELECT LISTAGG(value, ',' ON OVERFLOW TRUNCATE '.....' WITH COUNT) WITHIN GROUP (ORDER BY value)FROM (VALUES 'a', 'b', 'c') t(value);
listagg函数也可以用于分组相关的场景,例如:
SELECT id, LISTAGG(value, ',') WITHIN GROUP (ORDER BY o) csv_value FROM (VALUES (100, 1, 'a'), (200, 3, 'c'), (200, 2, 'b') ) t(id, o, value) GROUP BY id ORDER BY id; id | csv_value -----+------------- 100 | a 200 | b,c
- max_by(x, y)
select max_by(name,price) from fruit; -- peach
- max_by(x, y, n)
select max_by(name,price,2) from fruit;-- [peach, apple]
- min_by(x,y)
select min_by(name,price) from fruit;-- apple
- min_by(x, y, n)
select min_by(name,price,2) from fruit;-- [apple, peach]
- max(x)
select max(price) from fruit;-- 5
- max(x, n)
select max(price,2) from fruit; -- [5, 2]
- min(x)
select min(price) from fruit;-- 2
- min(x, n)
select min(price,2) from fruit;-- [2, 5]
- sum(T, x)
描述:对输入字段x求和,T为数值类型,如int,double,interval day to second等。
select sum(price) from fruit;-- 7
- regr_avgx(T independent, T dependent) → double
描述:计算回归线的自变量(expr2)的平均值,去掉了空对(expr1, expr2)后,等于AVG(expr2)。
create table sample_collection(id int,time_cost int,weight decimal(5,2)); insert into sample_collection values (1,5,86.38), (2,10,281.17), (3,15,89.91), (4,20,17.5), (5,25,88.76), (6,30,83.94), (7,35,44.26), (8,40,17.4), (9,45,5.6), (10,50,145.68); select regr_avgx(time_cost,weight) from sample_collection; _col0 ------------------- 86.06000000000002 (1 row)
- regr_avgy(T independent, T dependent) → double
描述:计算回归线的因变量(expr1)的平均值,去掉了空对(expr1, expr2)后,等于AVG(expr1)。
select regr_avgy(time_cost,weight) from sample_collection; _col0 ------- 27.5 (1 row)
- regr_count(T independent, T dependent) → double
select regr_count(time_cost,weight) from sample_collection; _col0 ------- 10 (1 row)
- regr_r2(T independent, T dependent) → double
select regr_r2(time_cost,weight) from sample_collection; _col0 -------------------- 0.1446739237728169 (1 row)
- regr_sxx(T independent, T dependent) → double
描述:返回值等于REGR_COUNT(expr1, expr2) * VAR_POP(expr2)。
select regr_sxx(time_cost,weight) from sample_collection; _col0 -------------------- 59284.886600000005 (1 row)
- regr_sxy(T independent, T dependent) → double
描述:返回值等于REGR_COUNT(expr1, expr2) * COVAR_POP(expr1, expr2)。
select regr_sxy(time_cost,weight) from sample_collection; _col0 ---------- -4205.95 (1 row)
- regr_syy(T independent, T dependent) → double
描述:返回值等于REGR_COUNT(expr1, expr2) * VAR_POP(expr1)。
select regr_syy(time_cost,weight) from sample_collection; _col0 -------- 2062.5 (1 row)
Bitwise聚合函数
Map聚合函数
- histogram(x) -> map(K, bigint)
select histogram(x),histogram(y) from (values (15,17),(15,18),(15,19),(15,20)) as t(x,y);-- {15=4},{17=1, 18=1, 19=1, 20=1}
- map_agg(key, value) -> map(K, V)
描述:返回一个由输入字段key和输入字段value为键值对的map。
select map_agg(name,price) from fruit;-- {apple=2, peach=5}
- map_union(x(K, V)) -> map(K, V)
描述:返回所有输入map的并集。如果一个key值在输入集中出现多次,对应的value取输入集中的key对应的任意值。
select map_union(x) from (values (map(array['banana'],array[10.0])),(map(array['apple'],array[7.0]))) as t(x);-- {banana=10.0, apple=7.0} select map_union(x) from (values (map(array['banana'],array[10.0])),(map(array['banana'],array[7.0]))) as t(x);-- {banana=10.0}
- multimap_agg(key, value) -> map(K, array(V))
描述:返回一个由输入key、value键值对组成的多重映射map。每个key可以对应多个value。
select multimap_agg(key, value) from (values ('apple',7),('apple',8),('apple',8),('lemon',5) ) as t(key,value); - {apple=[7, 8, 8], lemon=[5]}
近似值聚合函数
- approx_median(x) → bigint
select approx_median(price) from fruit; -- 10.0
- approx_distinct(x) → bigint
描述:该函数返回类型为bigint,它提供了count(distinct x)的近似计数。如果所有输入都是null值,则返回0。
此函数所有可能的值相对于正确的值的误差服从近似正态分布,其标准差为2.3%。它不保证任何特定输入集误差的上限。
select approx_distinct(price) from fruit; -- 2
- approx_distinct(x, e)→ bigint
描述:该函数返回类型为bigint,它提供了count(distinct x)的近似计数。如果所有输入都是null值,则返回0。
此函数所有可能的值相对于正确的值的误差服从近似正态分布,其标准差应小于e。它不保证任何特定输入集的误差的上限。
当前该函数的实现中,e的取值范围为[0.0040625,0.26000]。
select approx_distinct(weight,0.0040625) from sample_collection; -- 10 select approx_distinct(weight,0.26) from sample_collection; -- 8
- approx_most_frequent(buckets, value, capacity) → map<[same as value], bigint>
描述:近似统计出前buckets个最频繁出现的元素。函数统计高频值时,采用近似估算的方式使用的内存更少。capacity值越大,结果越精确,但消耗的内存也更多。该函数的返回结果是一个map,map的键值对为高频值及对应的频次。
SELECT approx_most_frequent(3, x, 15) FROM (values 'A', 'B', 'A', 'C', 'A', 'B', 'C', 'D', 'E') t(x); -- {A=3, B=2, C=2} SELECT approx_most_frequent(3, x, 100) FROM (values 1, 2, 1, 3, 1, 2, 3, 4, 5) t(x); -- {1=3, 2=2, 3=2}
分位数,常用的有二分位数,四分位数,十分位数,百分位数等,意味将输入集合均分为对应等份,然后找到大约位于该位置的数值。比如approx_percentile(x, 0.5)就是找到大约位于x值排序后大约50%位置的值,也就是二分位数。
- approx_percentile(x, percentage)→[same as x]
描述:根据给定的百分比,返回对应的近似百分位数。这个百分比的值对于所有输入的行来说必须是0到1之间的一个常量。
select approx_percentile(x, 0.5) from ( values (2),(3),(7),(8),(9)) as t(x); --7
- approx_percentile(x, percentages)→ array<[same as x]>
描述:以给定的百分比数组中的每个百分比,返回所有输入字段x值的近似百分位数。这个百分比数组中的每个值对于所有输入的行来说必须是0到1之间的一个常量。
select approx_percentile(x, array[0.1,0.2,0.3,0.5]) from ( values (2),(3),(7),(8),(9)) as t(x); --[2, 3, 3, 7]
- approx_percentile(x, w, percentage)→array<[same as x]>
描述:按照百分比percentage,返回所有x输入值的近似百分位数。每一项的权重值为w且必须为正数。x设置有效的百分位。percentage的值必须在0到1之间,并且所有输入行必须为常量。
select approx_percentile(x, 5,array[0.1,0.2,0.3,0.5]) from ( values (2),(3),(7),(8),(9)) as t(x); --[2, 3, 3, 7]
- approx_percentile(x, w, percentage, accuracy) →[same as x]
描述:按照百分比percentage,返回所有x输入值的近似百分位数。每一项的权重值为w且必须为正数。x设置有效的百分位。percentage的值必须在0到1之间,并且所有输入行必须为常量。其中,近似值的最大进度误差由accuracy指定。
select approx_percentile(x, 5,0.5,0.97) from ( values (2),(3),(7),(8),(9)) as t(x); --7
- approx_percentile(x, w, percentages)→[same as x]
描述:按照百分比数组中的每个百分比值,返回所有 x 输入值的近似百分位数。每一项的权重值为w且必须为正数。x设置有效的百分位。百分比数组中每个元素值必须在0到1之间,并且所有输入行必须为常量。
select approx_percentile(x,5, array[0.1,0.2,0.3,0.5]) from ( values (2),(3),(7),(8),(9)) as t(x); -- [2, 3, 3, 7]
以上approx_percentile函数也支持同参数集的percentile_approx函数。
- numeric_histogram(buckets, value, weight)
描述:按照buckets桶的数量,为所有的value计算近似直方图,每一项的宽度使用weight。本算法大体上基于。
Yael Ben-Haim and Elad Tom-Tov, "A streaming parallel decision tree algorithm", J. Machine Learning Research 11 (2010), pp. 849--872.
buckets必须是bigint。value和weight必须是数值类型。
select numeric_histogram(20,x,4) from ( values (2),(3),(7),(8),(9)) as t(x); _col0 ----------------------------------------------- {2.0=4.0, 3.0=4.0, 7.0=4.0, 8.0=4.0, 9.0=4.0} (1 row)
统计聚合函数
- corr(y,x)
select corr(y,x) from (values (1,5),(2,6),(3,7),(4,8)) as t(x,y);-- 1.0
- covar_pop(y, x)
select covar_pop(y,x) from (values (1,5),(2,6),(3,7),(4,8)) as t(x,y); --1.25
- covar_samp(y, x)
描述:返回输入值的样本协方差。
select covar_samp(y,x) from (values (1,5),(2,6),(3,7),(4,8)) as t(x,y);-- 1.6666666
- kurtosis(x)
描述:峰度又称峰态系数,表征概率密度分布曲线在平均值处峰值高低的特征数,即是描述总体中所有取值分布形态陡缓程度的统计量。直观看来,峰度反映了峰部的尖度。这个统计量需要与正态分布相比较。
定义上峰度是样本的标准四阶中心矩(standardized 4th central moment)。
随机变量的峰度计算方法为随机变量的四阶中心矩与方差平方的比值。
具体计算公式为:
select kurtosis(x) from (values (1),(2),(3),(4)) as t(x); -- -1.1999999999999993
- regr_intercept(y, x)
select regr_intercept(y,x) from (values (1,5),(2,6),(3,7),(4,8)) as t(x,y);-- 4.0
- regr_slope(y, x)
select regr_slope(y,x) from (values (1,5),(2,6),(3,7),(4,8)) as t(x,y);-- 1.0
- skewness(x)
select skewness(x) from (values (1),(2),(3),(4)) as t(x); -- 0.0
- stddev(x)
- stddev_pop(x)
select stddev_pop(x) from (values (1),(2),(3),(4)) as t(x);-- 1.118033988749895
- stddev_samp(x)
select stddev_samp(x) from (values (1),(2),(3),(4)) as t(x);-- 1.2909944487358056
- variance(x)
- var_pop(x)
select var_pop(x) from (values (1),(2),(3),(4)) as t(x);-- 1.25
- var_samp(x)
select var_samp(x) from (values (1),(2),(3),(4)) as t(x);-- 1.6666666666666667
Lambda聚合函数
reduce_agg(inputValue T, initialState S, inputFunction(S, T, S), combineFunction(S, S, S))
每个非空输入值将调用inputFunction。除了获取输入值之外,inputFunction还获取当前状态,最初为initialState,然后返回新状态。将调用CombineFunction将两个状态合并为一个新状态。 返回最终状态。
SELECT id, reduce_agg(value, 0, (a, b) -> a + b, (a, b) -> a + b) FROM ( VALUES (1, 3), (1, 4), (1, 5), (2, 6), (2, 7) ) AS t(id, value) GROUP BY id; -- (1, 12) -- (2, 13) SELECT id, reduce_agg(value, 1, (a, b) -> a * b, (a, b) -> a * b) FROM ( VALUES (1, 3), (1, 4), (1, 5), (2, 6), (2, 7) ) AS t(id, value) GROUP BY id; -- (1, 60) -- (2, 42)
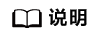
状态值必须是 boolean、integer、 floating-point或date、time、interval。