Flink SQL逻辑开发建议
在aggregate和join等操作前将数据过滤来减少计算的数据量
提前过滤可以减少在shuffle阶段前的数据量,减少网络IO,从而提升查询效率。
比如在表join前先过滤数据比在ON和WHERE时过滤可以有效较少join数据量。因为执行顺序从发生shuffle再filter变成了先发生filter再shuffle。
【示例】优化后将谓词条件A.userid>10提前到了子查询语句中,减少了shuffle的数据量:
- 优化前SQL:
select... from A join B on A.key = B.key where A.userid > 10 and B.userid < 10 and A.dt='20120417' and B.dt='20120417';
- 优化后SQL:
select ... from ( select ... from A where dt='201200417' and userid > 10 )a join ( select ... from B where dt='201200417' and userid < 10 )b on a.key = b.key;
慎用正则表达式函数REGEXP
正则表达式是非常耗时的操作,对比加减乘除通常有百倍的性能开销,而且正则表达式在某些极端情况下可能会进入无限循环,导致作业阻塞。推荐首先使用LIKE。正则函数包括:
- REGEXP
- REGEXP_EXTRACT
- REGEXP_REPLACE
【示例】
- 使用正则表达式:
SELECT * FROM table WHERE username NOT REGEXP "test|ceshi|tester'
- 使用like模糊查询:
SELECT * FROM table WHERE username NOT LIKE '%test%' AND username NOT LIKE '%ceshi%' AND username NOT LIKE '%tester%'
UDF嵌套不可过长
多个UDF嵌套时表达式长度很长,Flink优化生成的代码超过64KB导致编译错误。建议UDF嵌套不超过6个。
【示例】UDF嵌套:
SELECT SUM(get_order_total(order_id)) FROM orders WHERE customer_id = ( SELECT customer_id FROM customers WHERE customer_name = get_customer_name('John Doe') )
聚合函数中case when语法改写成filter语法
在聚合函数中,FILTER是更符合SQL标准用于过滤的语法,并且能获得更多的性能提升。FILTER是用于聚合函数的修饰符,用于限制聚合中使用的值。
【示例】在某些场景下需要从不同维度来统计UV,如Android中的UV,iPhone中的UV,Web中的UV和总UV,这时可能会使用如下CASE WHEN语法。
- 修改前:
SELECT day, COUNT(DISTINCT user_id) AS total_uv, COUNT(DISTINCT CASE WHEN flag IN (android', "iphone'") THEN user_id ELSE NULL END) AS app_uv, COUNT(DISTINCT CASE WHEN flag IN(wap', 'other') THEN user_id ELSE NULL END) AS web_uv FROM T GROUP BY day
- 修改后:
SELECT day, COUNT(DISTINCT user_id) AS total_uv, COUNT(DISTINCT user_id) FILTER (WHERE flag IN ('android', 'iphone')) AS app_uv, COUNT(DISTINCT user_id) FILTER(WHERE flag IN ('wap', 'other'))AS web_uv FROM T GROUP BY day
Flink SQL优化器可以识别相同的distinct key上的不同过滤器参数。例如示例中三个COUNT DISTINCT都在user_id列上。Flink可以只使用一个共享状态实例,而不是三个状态实例,以减少状态访问和状态大小,在某些工作负载下可以获得显著的性能提升。
拆分distinct聚合优化聚合中数据倾斜
通过两阶段聚合能消除常规的数据倾斜,但是处理distinct聚合时性能并不好。因为即使启动了两阶段聚合,distinct key也不能combine消除重复值,累加器中仍然包含所有的原始记录。
可以将不同的聚合(例如 COUNT(DISTINCT col))分为两个级别:
第一次聚合由group key和额外的bucket key进行shuffle。bucket key是使用HASH_CODE(distinct_key) % BUCKET_NUM计算的,BUCKET_NUM默认为1024,可以通过table.optimizer.distinct-agg.split.bucket-num选项进行配置。
第二次聚合是由原始group key进行shuffle,并使用SUM聚合来自不同buckets的COUNT DISTINCT值。由于相同的distinct key将仅在同一bucket中计算,因此转换是等效的。bucket key充当附加group key的角色,以分担group key中热点的负担。bucket key使Job具有可伸缩性来解决不同聚合中的数据倾斜/热点。
【示例】
- 资源文件配置:
table.optimizer.distinct-agg.split.enabled: true table.optimizer.distinct-agg.split.bucket-num: 1024
- 查询今天有多少唯一用户登录:
SELECT day, COUNT(DISTINCT user_id) FROM T GROUP BY day
- 自动改写查询:
SELECT day, SUM(cnt) FROM( SELECT day, COUNT(DISTINCT user_id) as cnt FROM T GROUP BY day, MOD(HASH_CODE(user_id), 1024) ) GROUP BY day
多流join场景建议join字段设置为主键
如果join字段不为主键,会导致Flink shuffle task按照hash进行数据处理,导致在Flink中无法保序。同时状态后端中同一个join key字段会保留多份,join时会产生笛卡尔积。
比如A表字段为“id, field1”,B表字段为“id, field2”。A表和B表根据“id”进行join,A表有历史数据(1, a1),B表有历史数据(1, b1)。当A表发生变化(1, a1)>(1, a2),同时B表发生变化(1, b1)>(1, b2)时,join结果如下,且join结果的顺序无法保证。
1, a1, b1 1, a2, b1 1, a1, b2 1, a2, b2
- 优化前SQL:
create table t1 ( id int, field1 string ) with( ...... ); create table t1 ( id int, field2 string ) with( ...... ); select t1.id, t1.field1, t2.field2 from t1 left join t2 on t1.id = t2.id;
- 优化后SQL:
create table t1 ( id int, field1 string, primary key (id) not enforced ) with( ...... ); create table t1 ( id int, field2 string, primary key (id) not enforced ) with( ...... ); select t1.id, t1.field1, t2.field2 from t1 left join t2 on t1.id = t2.id;
多表join场景且join key是联合主键时select字段要显示添加联合主键所有字段
如果不显示select联合主键所有字段,join算子会丢弃部分主键,导致join spec为NoUniqueKey。
- 优化前SQL:
create table table1( uuid varchar(20), name varchar(10), age int, ts timestamp, primary key (uuid) not enforced ) with ( 'connector' = 'datagen', 'rows-per-second' = '1' ); create table table2( uuid varchar(20), name varchar(10), age int, ts timestamp, primary key (uuid, name) not enforced ) with ( 'connector' = 'datagen', 'rows-per-second' = '1' ); create table print( uuid varchar(20), name varchar(10), age int, ts timestamp ) with ('connector' = 'print'); insert into print select t1.uuid, t1.name, t2.age, t2.ts from table1 t1 join table2 t2 on t1.uuid = t2.uuid;
图1 join spec为NoUniqueKey
- 优化后SQL:
create table table1( uuid varchar(20), name varchar(10), age int, ts timestamp, primary key (uuid) not enforced ) with ( 'connector' = 'datagen', 'rows-per-second' = '1' ); create table table2( uuid varchar(20), name varchar(10), age int, ts timestamp, primary key (uuid, name) not enforced ) with ( 'connector' = 'datagen', 'rows-per-second' = '1' ); create table print( uuid varchar(20), name varchar(10), name1 varchar(10), age int, ts timestamp ) with ('connector' = 'print'); insert into print select t1.uuid, t1.name, t2.name as name1, t2.age, t2.ts from table1 t1 join table2 t2 on t1.uuid = t2.uuid;
图2 优化后
多表left join场景下关联键发生改变使用雪花模型代替星型模型
多表left join关联键发生更新时会发生数据乱序,建议右表先关联成一个view,然后再与左表关联。
关联键group_id改变导致“-D”和“+I”乱序,下游根据user_id哈希时虽然进入同一并行度,但是“+I”消息先到,“-D”消息后到,最终写入宽表时记录就会被删除。
- 优化前SQL:
select... from t1 left join t2 on t2.user_id = t1.user_id left join t10 on t10.user_id = t1.user_id left join t11 on t11.group_id = t10.group_id left join t12 on t12.user_id = t1.user_id
- 优化后SQL:
create view tmp_view as( select .. from t10 left join t11 on t11.group_id = t10.group_id ); select... from t1 left join t2 on t2.user_id = t1.user_id left join tmp_view on tmp_view.user_id = t1.user_id left join t12 on t12.user_id = t1.user_id
多表left join时建议lookup join在所有双流join后
多表left join时建议lookup join在所有双流join后,否则下游有left join LATERAL TABLE时会发生乱序。
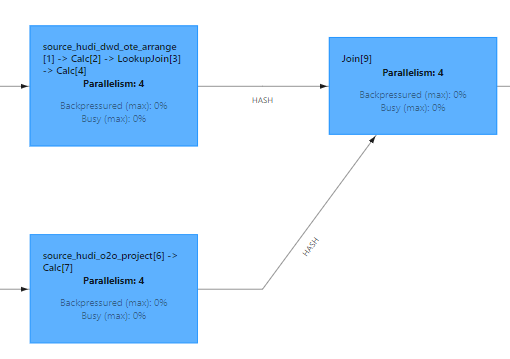
虽然左表已经定义主键,但是经过lookup join后下游left join时无法推断左流主键,导致左流所有历史数据都存储在状态,右流数据到达后会从最新的状态开始依次回撤左流状态中的每一条数据,经过LATERAL TABLE每一条source数据又与lateral table自关联,数据乱序。
查看打印结果可以看到连续多条“-D”消息,并且最后一条数据错误,因此建议lookup join放在双流join后执行。
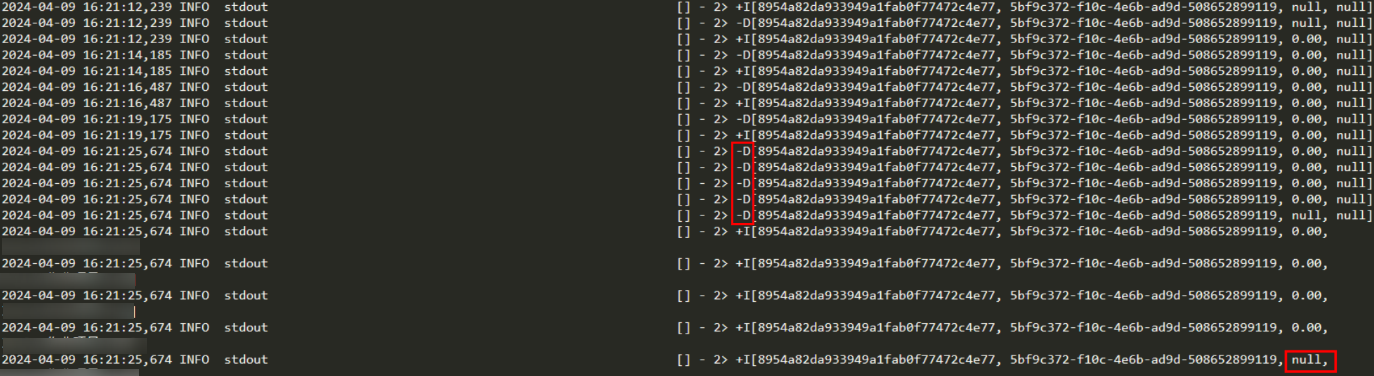
- 优化前SQL:
select... from t1 left join t2 FOR SYSTEM_TIME AS OF t1.proctime AS t21 on t21.id = t1.id left join t3 on t3.id = t1.id left join LATERAL TABLE(udtf()) AS t4(res1,res2.res3,res4) on true
- 优化后SQL:
select... from t1 left join t3 on t3.id = t1.id left join t2 FOR SYSTEM_TIME AS OF t1.proctime AS t21 on t21.id = t1.id left join LATERAL TABLE(udtf()) AS t4(res1,res2.res3,res4) on true
使用char数据类型时指定精度或者改用string类型
使用“cast(id as char)”数据类型转换时,结果只截取第一位,导致数据错误。如果转换字段正好是主键字段则会丢失大量数据。
配置“table.exec.legacy-cast-behaviour=ENABLED”也可以解决转换发生错误的问题,但是不建议使用。
在Flink 1.15之前,可以通过将“table.exec.legacy-cast-behaviour”设置为“enabled”来启用旧版本的类型转换行为。但在Flink 1.15及之后版本中,默认情况下该标志被禁用,将导致以下行为:
- 转换为CHAR/VARCHAR/BINARY/VARBINARY时禁用修剪/填充操作。
- CAST操作永远不会失败,而是返回NULL,类似于TRY_CAST,但不会推断正确的类型。
- 对于某些转换为CHAR/VARCHAR/STRING的格式化操作,结果可能略有不同。
我们不建议使用此标志,并强烈建议新项目保持禁用该标志并使用新的类型转换行为。该标志将在未来的Flink版本中被移除。
- 优化前SQL:
select cast(id as char) as id, ... from t1
- 优化后SQL:
select cast(id as string) as id, ... from t1
多个Flink作业或者insert into语句写同一张Gauss for MySQL时建议过滤回撤数据
当有多个Flink作业写同一张MySQL表时,其中一个Flink作业发送回撤数据(-D、-U)到目标表删除整行数据,再插入本次更新的数据,导致其他作业写入的字段全部丢失。
- 优化前SQL:
create table source-A( id, user_id )with( 'connector' = 'kafka' ); create table source-B( id, org_id )with( 'connector' = 'kafka' ); create table sink-A( id, user_id )with( 'connector' = 'jdbc' 'url' = 'jdbc:mysql://****', 'table-name' = 'sink-table' ); create table sink-B( id, org_id )with( 'connector' = 'jdbc' 'url' = 'jdbc:mysql://****', 'table-name' = 'sink-table' ); insert into sink-A select id,user_id from source-A; insert into sink-B select id,org_id from source-B;
- 优化后SQL:
create table source-A( id, user_id )with( 'connector' = 'kafka' ); create table source-B( id, org_id )with( 'connector' = 'kafka' ); create table sink-A( id, user_id )with( 'connector' = 'jdbc' 'url' = 'jdbc:mysql://****', 'table-name' = 'sink-table', 'filter.record.enabled' = 'true' ); create table sink-B( id, org_id )with( 'connector' = 'jdbc' 'url' = 'jdbc:mysql://****', 'table-name' = 'sink-table', 'filter.record.enabled' = 'true' ); insert into sink-A select id,user_id from source-A; insert into sink-B select id,org_id from source-B;