What Is GaussDB(DWS)?
GaussDB(DWS) is an online data analysis and processing database built on the Huawei Cloud infrastructure and platform. It offers scalable, ready-to-use, and fully managed analytical database services, and is compatible with ANSI/ISO SQL92, SQL99, and SQL 2003 syntax. Additionally, GaussDB(DWS) is interoperable with other database ecosystems such as PostgreSQL, Oracle, Teradata, and MySQL. This makes it a competitive option for petabyte-scale big data analytics across diverse industries.
GaussDB(DWS) comes in the following types: standard data warehouse (DWS 2.0 and DWS 3.0) and hybrid data warehouse, helping you build a data warehouse leading in the industry in terms of enterprise-level kernels, real-time analysis, collaborative computing, convergent analysis, and cloud native. For details, see Data Warehouse Types.
- Standard Data Warehouse (DWS 2.0): Oriented to data analysis scenarios, DWS 2.0 provides enterprise-level data warehouse services with high performance, high scalability, high reliability, high security, and easy O&M. It is capable of data analysis at a scale of 2,048 nodes and 20 petabytes of data.
- Standard data warehouse (DWS 3.0): The DWS 3.0 data warehouse is designed with a cloud native architecture that separates storage and compute. It also features hierarchical auto scaling for computing and storage, as well as multi-logical cluster shared storage technology (Virtual Warehouse or VW). These capabilities allow for computing isolation and concurrent expansion to handle varying loads, making it an ideal choice for OLAP analysis scenarios.
- Hybrid data warehouse: It provides high-concurrency, high-performance, and low-latency transaction processing capabilities based on large-scale data query and analysis capabilities. It is suitable for hybrid transaction/analytical processing (HTAP). A database can be used for both production and analysis.
GaussDB(DWS) is widely used in domains such as finance, Internet of Vehicles (IoV), government and enterprise, e-commerce, energy, and telecom. It has been listed in the Gartner Magic Quadrant for Data Management Solutions for Analytics for two consecutive years. Unlike conventional data warehouses, GaussDB(DWS) is more cost-effective and has large-scale scalability and enterprise-level reliability.
In addition, GaussDB(DWS) can be deployed on physical machines. For details, see Physical Machine Deployment.
Logical Cluster Architecture
Figure 1 shows the logical architecture of a GaussDB(DWS) cluster. For details about the instance, see Table 1.
Name |
Function |
Description |
---|---|---|
Cluster Manager (CM) |
Cluster Manager. It manages and monitors the running status of functional units and physical resources in the distributed system, ensuring system stability. |
The CM consists of CM Agent, OM Monitor, and CM Server.
GaussDB(DWS) provides the primary/standby CM Server solution to ensure system HA. CM Agent connects to the primary CM Server. If the primary CM Server is faulty, the standby CM Server is promoted to primary to prevent a single point of failure (SPOF). |
Global Transaction Manager (GTM) |
Generates and maintains the globally unique information, such as the transaction ID, transaction snapshot, and timestamp. |
The cluster includes only one pair of GTMs: one primary GTM and one standby GTM. |
Workload Manager (WLM) |
Workload Manager. It controls allocation of system resources to prevent service congestion and system crash resulting from excessive workload. |
You do not need to specify names of hosts where WLMs are to be deployed, because the installation program automatically installs a WLM on each host. |
Coordinator (CN) |
A CN receives access requests from applications, and returns execution results to the client; splits tasks and allocates task fragments to different DNs for parallel processing. |
CNs in a cluster have equivalent roles and return the same result for the same DML statement. Load balancers can be added between CNs and applications to ensure that CNs are transparent to applications. If a CN is faulty, the load balancer automatically connects the application to the other CN. For details, see Associating and Disassociating ELB. CNs need to connect to each other in the distributed transaction architecture. To reduce heavy load caused by excessive threads on GTMs, no more than 10 CNs should be configured in a cluster. GaussDB(DWS) handles the global resource load in a cluster using the Central Coordinator (CCN) for adaptive dynamic load management. When the cluster is started for the first time, the CM selects the CN with the smallest ID as the CCN. If the CCN is faulty, CM replaces it with a new one. |
Datanode (DN) |
A DN stores data in row-store, column-store, or hybrid mode, executes data query tasks, and returns execution results to CNs. |
There are multiple DNs in the cluster. Each DN stores part of data. GaussDB(DWS) provides DN high availability: active DN, standby DN, and secondary DN. The working principles of the three are as follows:
The secondary DN serves exclusively as a backup, never ascending to active or standby status in case of faults. It conserves storage by only holding Xlog data transferred from the new active DN and data replicated during original active DN failures. This efficient approach saves one-third of the storage space compared to conventional tri-backup methods. |
Storage |
Functions as the server's local storage resources to store data permanently. |
- |
DNs in a cluster store data on disks. Figure 2 describes the objects on each DN and the relationships among them logically.
- A database manages various data objects and is isolated from other databases.
- A datafile segment stores data in only one table. A table containing more than 1 GB of data is stored in multiple data file segments.
- A table belongs only to one database.
- A block is the basic unit of database management, with a default size of 8 KB.
Data can be distributed in replication, round-robin, or hash mode. You can specify the distribution mode during table creation.
Physical Architecture of a Cluster
GaussDB(DWS) supports storage-compute integration (2.0) and storage-compute decoupling (3.0).
In the storage and compute integrated architecture, data is stored on local disks of DNs. In the storage-compute decoupling architecture, local DN disks are used only for data cache and metadata storage, and user data is stored on OBS. You can select an architecture as required.
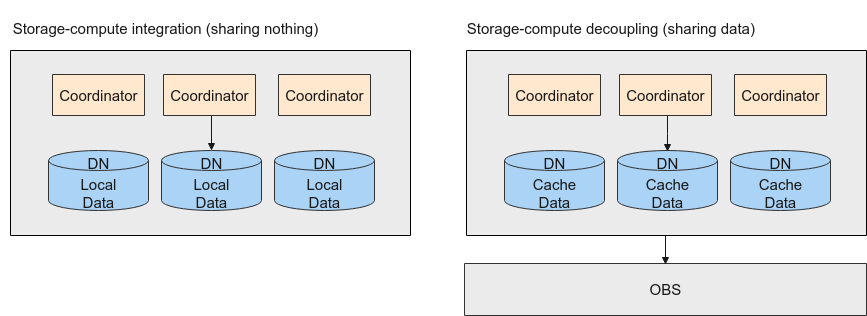
Storage-Compute Integration (2.0)
GaussDB(DWS) employs the shared-nothing architecture and the massively parallel processing (MPP) engine, and consists of numerous independent logical nodes that do not share the system resources such as CPUs, memory, and storage. In such a system architecture, service data is separately stored on numerous nodes. Data analysis tasks are executed in parallel on the nodes where data is stored. The massively parallel data processing significantly improves response speed.
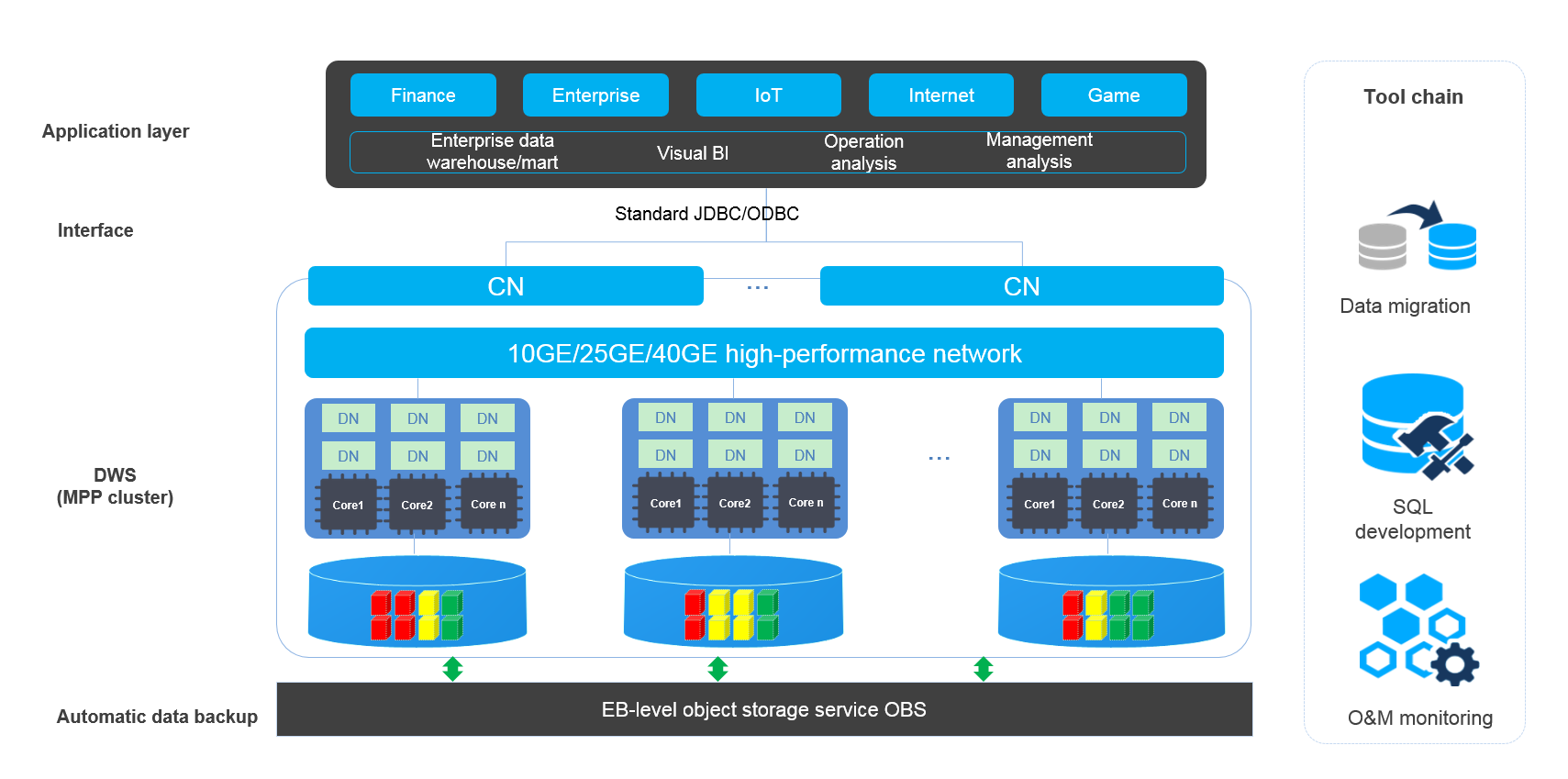
- Application layer
Data loading tools, extract, transform, and load (ETL) tools, business intelligence (BI) tools, as well as data mining and analysis tools, can be integrated with GaussDB(DWS) through standard APIs. GaussDB(DWS) is compatible with the PostgreSQL ecosystem, and the SQL syntax is compatible with Oracle, MySQL, and Teradata. Applications can be smoothly migrated to GaussDB(DWS) with few changes.
- API
Applications can connect to GaussDB(DWS) through standard JDBC and ODBC.
- GaussDB(DWS)
A GaussDB(DWS) cluster contains nodes of the same flavor in the same subnet. These nodes jointly provide services. Datanodes (DNs) in a cluster store data on disks. CNs, or Coordinators, receive access requests from the clients and return the execution results. They also split and distribute tasks to the Datanodes (DNs) for parallel execution.
- Automatic data backup
Cluster snapshots can be automatically backed up to the EB-level Object Storage Service (OBS), which facilitates periodic backup of the cluster during off-peak hours, ensuring data recovery after a cluster exception occurs.
A snapshot is a complete backup of GaussDB(DWS) at a specified time point. It records all configuration data and service data of the cluster at the specified moment.
- Tool chain
The parallel data loading tool General Data Service (GDS), SQL syntax migration tool Database Schema Convertor (DSC), and SQL development tool Data Studio are provided. The cluster O&M can be monitored on a console.
Storage-Compute Decoupling Architecture (3.0)
The newly released DWS 3.0 version provides resource pooling, massive storage, and the MPP architecture with decoupled compute and storage. This enables high elasticity, real-time data import and sharing, and lake warehouse integration.
DWS 3.0 allows for separate scaling of compute and storage resources through decoupled compute and storage. Users can easily adjust their computing capabilities during peak and off-peak hours. Additionally, storage can be expanded limitlessly and paid for on-demand, allowing for quick and flexible responses to service changes while maintaining cost-effectiveness.
DWS 3.0 has the following advantages:
- Lakehouse: DWS 3.0 simplifies the maintenance and operation of an integrated lakehouse. It seamlessly integrates with DLI, supports automatic metadata import, accelerates external table queries, enables joined queries of internal and external tables, and allows for reading and writing of data lake formats, as well as easier data import.
- Real-time write: DWS 3.0 provides the H-Store storage engine which optimizes real-time data writes and supports high-throughput real-time batch writes and updates.
- High elasticity: Scaling compute resources and using on-demand storage can result in significant cost savings. Historical data does not need to be migrated to other storage media, enabling one-stop data analysis for industries such as finance and Internet.
- Data sharing: Multiple loads share one copy of data in real time, while the computing resources are isolated. Multiple writes and reads are supported.
- Superb scalability
- Logical clusters, known as Virtual Warehouses (VWs), can be expanded concurrently based on service requirements.
- Data is shared among multiple VWs in real-time, eliminating the need for data duplication.
- Multiple VWs enhance throughput and concurrency while providing excellent read/write and load isolation.
- Lakehouse
- Seamless hybrid query across data lakes and data warehouses
- In data lake analysis, you can enjoy the ultimate performance and precise control of data warehouses.
Comparison Between DWS 3.0 and DWS 2.0
Version |
DWS 2.0 |
DWS 3.0 |
||
---|---|---|---|---|
Storage medium |
Data is stored on local disks of compute nodes. |
Column-store data is stored in Huawei Cloud OBS. Local disks are used as the query cache of OBS data. Row-store data is still stored in local disks of compute nodes. |
||
Advantage |
Data is stored locally on compute nodes, providing high performance. |
The architecture separates storage and compute, offering layered elasticity, on-demand storage use, rapid compute scaling, unlimited computing power, and capacity. Data stored on object storage reduces costs and multiple VWs support higher concurrency. Data sharing and lakehouse integration. |
Feedback
Was this page helpful?
Provide feedbackThank you very much for your feedback. We will continue working to improve the documentation.See the reply and handling status in My Cloud VOC.
For any further questions, feel free to contact us through the chatbot.
Chatbot