Help Center/
GaussDB/
Feature Guide(Centralized_3.x)/
Partitioned Table/
Partitioned Table Query Optimization/
Partition Pruning/
Dynamic Partition Pruning/
Dynamic Parameterized Path Pruning
Updated on 2024-05-20 GMT+08:00
Dynamic Parameterized Path Pruning
Dynamic parameterized path pruning is supported in the following scenarios:
- Supported partitioning levels: level-1 partition and level-2 partition
- Supported partitioning types: range partitioning, interval partitioning, hash partitioning, and list partitioning.
- Supported operator types: index scan, index-only scan, and bitmap scan.
- Supported expression types: comparison expression (<, <=, =, >=, >) and logical expression.
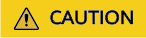
Dynamic parameterized path pruning does not support subquery expressions, STABLE and VOLATILE functions, cross-QueryBlock parameterized paths, BitmapOr operator, or BitmapAnd operator.
- Typical scenarios where dynamic parameterized path pruning is supported are as follows:
- Comparison expressions
-- Create partitioned tables and indexes. gaussdb=# CREATE TABLE t1 (c1 INT, c2 INT) PARTITION BY RANGE (c1) ( PARTITION p1 VALUES LESS THAN(10), PARTITION p2 VALUES LESS THAN(20), PARTITION p3 VALUES LESS THAN(MAXVALUE) ); gaussdb=# CREATE TABLE t2 (c1 INT, c2 INT) PARTITION BY RANGE (c1) ( PARTITION p1 VALUES LESS THAN(10), PARTITION p2 VALUES LESS THAN(20), PARTITION p3 VALUES LESS THAN(MAXVALUE) ); gaussdb=# CREATE INDEX t1_c1 ON t1(c1) LOCAL; gaussdb=# CREATE INDEX t2_c1 ON t2(c1) LOCAL; gaussdb=# CREATE INDEX t1_c2 ON t1(c2) LOCAL; gaussdb=# CREATE INDEX t2_c2 ON t2(c2) LOCAL; gaussdb=# EXPLAIN (VERBOSE ON, COSTS OFF) SELECT * FROM t2 JOIN t1 ON t1.c1 = t2.c2; QUERY PLAN ------------------------------------------------------------- Hash Join Output: t2.c1, t2.c2, t1.c1, t1.c2 Hash Cond: (t2.c2 = t1.c1) -> Partition Iterator Output: t2.c1, t2.c2 Iterations: 3 -> Partitioned Seq Scan on public.t2 Output: t2.c1, t2.c2 Selected Partitions: 1..3 -> Hash Output: t1.c1, t1.c2 -> Partition Iterator Output: t1.c1, t1.c2 Iterations: 3 -> Partitioned Seq Scan on public.t1 Output: t1.c1, t1.c2 Selected Partitions: 1..3 (17 rows) gaussdb=# EXPLAIN (VERBOSE ON, COSTS OFF) SELECT * FROM t2 JOIN t1 ON t1.c1 < t2.c2; QUERY PLAN ------------------------------------------------------------- Nested Loop Output: t2.c1, t2.c2, t1.c1, t1.c2 -> Partition Iterator Output: t2.c1, t2.c2 Iterations: 3 -> Partitioned Seq Scan on public.t2 Output: t2.c1, t2.c2 Selected Partitions: 1..3 -> Partition Iterator Output: t1.c1, t1.c2 Iterations: PART -> Partitioned Index Scan using t2_c1 on public.t1 Output: t1.c1, t1.c2 Index Cond: (t1.c1 < t2.c2) Selected Partitions: 1..3 (ppi-pruning) (15 rows) gaussdb=# EXPLAIN (VERBOSE ON, COSTS OFF) SELECT * FROM t2 JOIN t1 ON t1.c1 > t2.c2; QUERY PLAN ------------------------------------------------------------- Nested Loop Output: t2.c1, t2.c2, t1.c1, t1.c2 -> Partition Iterator Output: t2.c1, t2.c2 Iterations: 3 -> Partitioned Seq Scan on public.t2 Output: t2.c1, t2.c2 Selected Partitions: 1..3 -> Partition Iterator Output: t1.c1, t1.c2 Iterations: PART -> Partitioned Index Scan using t2_c1 on public.t1 Output: t1.c1, t1.c2 Index Cond: (t1.c1 > t2.c2) Selected Partitions: 1..3 (ppi-pruning) (15 rows)
- Logical expressions
gaussdb=# EXPLAIN (VERBOSE ON, COSTS OFF) SELECT * FROM t2 JOIN t1 ON t1.c1 = t2.c2 AND t1.c2 = 2; QUERY PLAN ------------------------------------------------------------- Hash Join Output: t2.c1, t2.c2, t1.c1, t1.c2 Hash Cond: (t2.c2 = t1.c1) -> Partition Iterator Output: t2.c1, t2.c2 Iterations: 3 -> Partitioned Seq Scan on public.t2 Output: t2.c1, t2.c2 Selected Partitions: 1..3 -> Hash Output: t1.c1, t1.c2 -> Partition Iterator Output: t1.c1, t1.c2 Iterations: 3 -> Partitioned Bitmap Heap Scan on public.t1 Output: t1.c1, t1.c2 Recheck Cond: (t1.c2 = 2) Selected Partitions: 1..3 -> Partitioned Bitmap Index Scan on t1_c2 Index Cond: (t1.c2 = 2) (20 rows)
- Comparison expressions
- Typical scenarios where dynamic parameterized path pruning is not supported are as follows:
- BitmapOr and BitmapAnd operators
gaussdb=# SET enable_seqscan=off; gaussdb=# EXPLAIN (VERBOSE ON, COSTS OFF) SELECT * FROM t2 JOIN t1 ON t1.c1 = t2.c2 OR t1.c1 = 2; QUERY PLAN ---------------------------------------------------------------- Nested Loop Output: t2.c1, t2.c2, t1.c1, t1.c2 -> Partition Iterator Output: t2.c1, t2.c2 Iterations: 3 -> Partitioned Seq Scan on public.t2 Output: t2.c1, t2.c2 Selected Partitions: 1..3 -> Partition Iterator Output: t1.c1, t1.c2 Iterations: 3 -> Partitioned Bitmap Heap Scan on public.t1 Output: t1.c1, t1.c2 Recheck Cond: ((t1.c1 = t2.c2) OR (t1.c1 = 2)) Selected Partitions: 1..3 -> BitmapOr -> Partitioned Bitmap Index Scan on t1_c1 Index Cond: (t1.c1 = t2.c2) -> Partitioned Bitmap Index Scan on t1_c1 Index Cond: (t1.c1 = 2) (20 rows)
- Implicit conversion
gaussdb=# CREATE TABLE t3(c1 TEXT, c2 INT); CREATE TABLE gaussdb=# EXPLAIN (VERBOSE ON, COSTS OFF) SELECT * FROM t1 JOIN t3 ON t1.c1 = t3.c1; QUERY PLAN ------------------------------------------------------------- Nested Loop Output: t1.c1, t1.c2, t3.c1, t3.c2 -> Seq Scan on public.t3 Output: t3.c1, t3.c2 -> Partition Iterator Output: t1.c1, t1.c2 Iterations: 3 -> Partitioned Index Scan using t1_c1 on public.t1 Output: t1.c1, t1.c2 Index Cond: (t1.c1 = (t3.c1)::bigint) Selected Partitions: 1..3 (11 rows)
- Functions
gaussdb=# EXPLAIN (VERBOSE ON, COSTS OFF) SELECT * FROM t1 JOIN t3 ON t1.c1 = LENGTHB(t3.c1); QUERY PLAN ------------------------------------------------------------- Nested Loop Output: t1.c1, t1.c2, t3.c1, t3.c2 -> Seq Scan on public.t3 Output: t3.c1, t3.c2 -> Partition Iterator Output: t1.c1, t1.c2 Iterations: 3 -> Partitioned Index Scan using t1_c1 on public.t1 Output: t1.c1, t1.c2 Index Cond: (t1.c1 = lengthb(t3.c1)) Selected Partitions: 1..3 (11 rows) -- Cleanup example gaussdb=# DROP TABLE t1; gaussdb=# DROP TABLE t2; gaussdb=# DROP TABLE t3;
- BitmapOr and BitmapAnd operators
Parent topic: Dynamic Partition Pruning
Feedback
Was this page helpful?
Provide feedbackThank you very much for your feedback. We will continue working to improve the documentation.See the reply and handling status in My Cloud VOC.
The system is busy. Please try again later.
For any further questions, feel free to contact us through the chatbot.
Chatbot