Incremental Model Training
What Is Incremental Training?
Incremental learning is a machine learning method that enables AI models to learn from new data without restarting the training process. It builds on existing knowledge, allowing the model to expand its capabilities and improve its performance over time.
Incremental learning allows for training on data in smaller chunks, reducing storage needs and alleviating resource constraints. It also conserves computing power and time, and lowers retraining costs.
Incremental training is ideal for these scenarios:
- Continuous data updates: It allows models to adapt to new data without retraining.
- Resource constraints: It is a more economical choice when retraining a model is too costly.
- Avoiding knowledge loss: It retains old knowledge while learning new information, preventing the model from forgetting what it has learned.
Incremental training is used in various fields, including natural language processing, computer vision, and recommendation systems. It makes AI systems more flexible and adaptable, allowing them to handle changing data in real-world environments.
Implementing Incremental Training in ModelArts Standard
The checkpoint mechanism enables incremental training.
During model training, training results (including but not limited to epochs, model weights, optimizer status, and scheduler status) are continuously saved. To add data and resume a training job, load a checkpoint and use the checkpoint information to initialize the training status. To do so, add reload ckpt to the code.
To incrementally train a model in ModelArts, configure the training output.
When creating a training job, set the data path to the training output, save checkpoints in this data path, and set Predownload to Yes. If you set Predownload to Yes, the system automatically downloads the checkpoint file in the training output data path to a local directory of the training container before the training job is started.
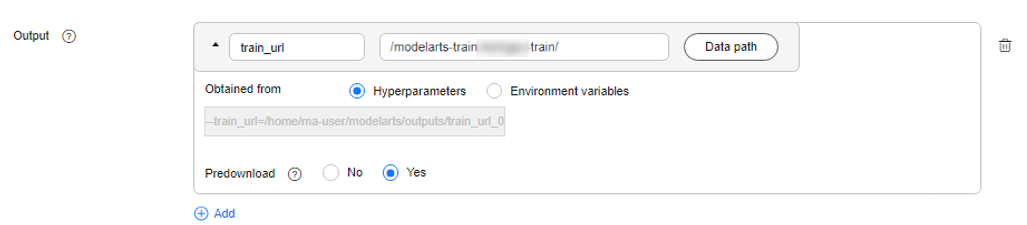
reload ckpt for PyTorch
- Use either of the following methods to save a PyTorch model.
- Save model parameters only.
state_dict = model.state_dict() torch.save(state_dict, path)
- Save the entire model (not recommended).
torch.save(model, path)
- Save model parameters only.
- Save the data generated during model training at regular intervals based on steps and time.
The data includes the network weight, optimizer weight, and epoch, which will be used to resume the interrupted training.
checkpoint = { "net": model.state_dict(), "optimizer": optimizer.state_dict(), "epoch": epoch } if not os.path.isdir('model_save_dir'): os.makedirs('model_save_dir') torch.save(checkpoint,'model_save_dir/ckpt_{}.pth'.format(str(epoch)))
- Check the complete code example below.
import os import argparse parser = argparse.ArgumentParser() parser.add_argument("--train_url", type=str) args, unparsed = parser.parse_known_args() args = parser.parse_known_args() # train_url is set to /home/ma-user/modelarts/outputs/train_url_0. train_url = args.train_url # Check whether there is a model file in the output path. If there is no file, the model will be trained from the beginning by default. If there is a model file, the CKPT file with the maximum epoch value will be loaded as the pre-trained model. if os.listdir(train_url): print('> load last ckpt and continue training!!') last_ckpt = sorted([file for file in os.listdir(train_url) if file.endswith(".pth")])[-1] local_ckpt_file = os.path.join(train_url, last_ckpt) print('last_ckpt:', last_ckpt) # Load the checkpoint. checkpoint = torch.load(local_ckpt_file) # Load the parameters that can be learned by the model. model.load_state_dict(checkpoint['net']) # Load optimizer parameters. optimizer.load_state_dict(checkpoint['optimizer']) # Obtain the saved epoch. The model will continue to be trained based on the epoch value. start_epoch = checkpoint['epoch'] start = datetime.now() total_step = len(train_loader) for epoch in range(start_epoch + 1, args.epochs): for i, (images, labels) in enumerate(train_loader): images = images.cuda(non_blocking=True) labels = labels.cuda(non_blocking=True) # Forward pass outputs = model(images) loss = criterion(outputs, labels) # Backward and optimize optimizer.zero_grad() loss.backward() optimizer.step() ... # Save the network weight, optimizer weight, and epoch during model training. checkpoint = { "net": model.state_dict(), "optimizer": optimizer.state_dict(), "epoch": epoch } if not os.path.isdir(train_url): os.makedirs(train_url) torch.save(checkpoint, os.path.join(train_url, 'ckpt_best_{}.pth'.format(epoch)))
Feedback
Was this page helpful?
Provide feedbackThank you very much for your feedback. We will continue working to improve the documentation.See the reply and handling status in My Cloud VOC.
For any further questions, feel free to contact us through the chatbot.
Chatbot