Training a Model
After the ExeML task is created, a model is trained for predictive analytics. You can publish the model as a real-time inference service.
Procedure
- On the ExeML page of the new version, click the name of the target project to view the execution status of the current workflow.
- On the predictive analytics phase, wait until the phase status changes from Running to Executed.
- Click
to view the training details, such as the label column, data type, accuracy, and evaluation result.
The example is a discrete value of binary classification. For details about the evaluation result parameters, see Table 1.
For details about the evaluation results generated for different data types of label columns, see Evaluation Results.
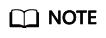
An ExeML project supports multiple rounds of training, and each round generates an AI application version. For example, the first training version is 0.0.1, and the next version is 0.0.2. The trained models can be managed by training version. After the trained model meets your requirements, deploy the model as a service.
Evaluation Results
The parameters in evaluation results vary depending on the training data type.
- Discrete values
The evaluation parameters include recall, precision, accuracy, and F1 score, which are described in the following table.
Table 1 Parameters in discrete value evaluation results Parameter
Description
Recall
Fraction of correctly predicted samples over all samples predicted as a class. It shows the ability of a model to distinguish positive samples.
Precision
Fraction of correctly predicted samples over all samples predicted as a class. It shows the ability of a model to distinguish negative samples.
Accuracy
Fraction of correctly predicted samples over all samples. It shows the general ability of a model to recognize samples.
F1 Score
Harmonic average of the precision and recall of a model. It is used to evaluate the quality of a model. A high F1 score indicates a good model.
- Continuous values
The evaluation parameters include Mean Absolute Error (MAE), Mean Squared Error (MSE), and Root Mean Squared Error (RMSE). The three error values represent a difference between a real value and a predicted value. During multiple rounds of modeling, a group of error values is generated for each round of modeling. Use these error values to determine the quality of a model. A smaller error value indicates a better model.
Feedback
Was this page helpful?
Provide feedbackThank you very much for your feedback. We will continue working to improve the documentation.See the reply and handling status in My Cloud VOC.
For any further questions, feel free to contact us through the chatbot.
Chatbot