Creating a Production Training Job
Model training continuously iterates and optimizes model weights. ModelArts training management allows you to create training jobs, view training status, and manage training versions. Through model training, you can test various combinations of model structures, data, and hyperparameters to obtain the optimal model structure and weight.
Create a production training job in either of the following ways:
- Use the ModelArts Standard console. For details, see the following sections.
- Use the ModelArts API to create a production training job. For details, see Using PyTorch to Create a Training Job (New-Version Training).
Prerequisites
- Data for training uploaded to an OBS directory.
- At least one empty folder in OBS for storing training output.
ModelArts does not support encrypted OBS buckets. When creating an OBS bucket, do not enable bucket encryption.
- Account not in arrears (paid resources required for training jobs).
- OBS directory and ModelArts in the same region.
- Access authorization configured. If it is not configured, configure it by referring to Configuring Access Authorization for ModelArts Standard.
- Training algorithm. For details, see Creating an Algorithm.
Procedure
To create a training job, follow these steps:
- Follow the steps in Accessing the Page for Creating a Training Job.
- Follow the steps in Configuring Basic Information.
- Select an algorithm type.
- Use an existing algorithm to create a training job by referring to Choosing an Algorithm Type (My Algorithm).
- Use a preset image to create a training job by referring to Choosing an Algorithm Type (Custom Algorithm).
- Use a custom image to create a training job by referring to Choosing a Boot Mode (Custom Image).
- Configure training parameters, including the input, output, hyperparameters, and environment variables. For details, see Configuring Training Parameters.
- Select a resource pool as needed. A dedicated resource pool is recommended for optimal performance. For details about the differences between dedicated and public resource pools, see Differences Between Dedicated Resource Pools and Public Resource Pools.
- Select a training mode when you use a preset MindSpore engine and Ascend resources. For details, see (Optional) Selecting a Training Mode.
- Add tags if you want to manage training jobs by group. For details, see (Optional) Adding Tags.
- Perform follow-up procedure. For details, see Follow-Up Operations.
Accessing the Page for Creating a Training Job
- Log in to the ModelArts console.
- In the navigation pane, choose .
- Click Create Training Job.
Configuring Basic Information
On the Create Training Job page, configure parameters.
Parameter |
Description |
---|---|
Name |
Job name, which is mandatory. The system automatically generates a name, which you can then rename according to the following rules.
|
Description |
Job description, which helps you learn about the job information in the training job list. |
Choosing an Algorithm Type (My Algorithm)
Set Algorithm Type to My algorithm and select an algorithm from the algorithm list. If no algorithm meets the requirements, you can create an algorithm. For details, see Creating an Algorithm.
Choosing an Algorithm Type (Custom Algorithm)
Parameter |
Description |
---|---|
Algorithm Type |
Select Custom algorithm. This parameter is mandatory. |
Boot Mode |
Select Preset image and select the preset image engine and engine version to be used by the training job. If you select Customize for the engine version, select a custom image from Image. |
Image |
This parameter is displayed and mandatory only when the preset image version is set to Customize.
You can set the container image path in either of the following ways:
|
Code Source |
Select a training code source.
|
Code Directory |
This parameter is available only when Code Source is set to OBS. Select the OBS directory where the training code file is stored. This parameter is mandatory.
|
Boot File |
Select the Python boot script of the training job in the code directory. This parameter is mandatory. ModelArts supports only the boot file written in Python. Therefore, the boot file must end with .py. |
Local Code Directory |
This parameter is available only when Code Source is set to OBS. Specify the local directory of a training container. When a training starts, the system automatically downloads the code directory to this directory. The default local code directory is /home/ma-user/modelarts/user-job-dir. This parameter is optional. |
Work Directory |
During training, the system automatically runs the cd command to execute the boot file in this directory. |
- The system automatically injects environment variables.
PATH=${MA_HOME}/anaconda/bin:${PATH} LD_LIBRARY_PATH=${MA_HOME}/anaconda/lib:${LD_LIBRARY_PATH} PYTHONPATH=${MA_JOB_DIR}:${PYTHONPATH}
- The selected boot file will be automatically started using Python commands. Ensure that the Python environment is correct. The PATH environment variable is automatically injected. Run the following commands to check the Python version for the training job:
export MA_HOME=/home/ma-user; docker run --rm {image} ${MA_HOME}/anaconda/bin/python -V docker run --rm {image} $(which python) -V
- The system automatically adds hyperparameters associated with the preset image.
Choosing a Boot Mode (Custom Image)
Parameter |
Description |
---|---|
Algorithm Type |
Select Custom algorithm. This parameter is mandatory. |
Boot Mode |
Select Custom image. This parameter is mandatory. |
Image |
Container image path. This parameter is mandatory.
You can set the container image path in either of the following ways:
|
Code Directory |
OBS directory where the training code file is stored. Configure this parameter only if your custom image does not contain training code.
|
User ID |
User ID for running the container. The default value 1000 is recommended. If the UID needs to be specified, its value must be within the specified range. The UID ranges of different resource pools are as follows:
|
Boot Command |
Command for booting an image. This parameter is mandatory.
When a training job is running, the boot command is automatically executed after the code directory is downloaded.
You can use semicolons (;) and ampersands (&&) to combine multiple commands. demo-code in the command is the last-level OBS directory where the code is stored. Replace it with the actual one.
NOTE:
To ensure data security, do not enter sensitive information, such as plaintext passwords. |
Local Code Directory |
This parameter is available only when Code Source is set to OBS. Specify the local directory of a training container. When a training starts, the system automatically downloads the code directory to this directory. The default local code directory is /home/ma-user/modelarts/user-job-dir. This parameter is optional. |
Work Directory |
During training, the system automatically runs the cd command to execute the boot file in this directory. |
For details about how to use custom images supported by training, see Boot Command Specifications for Custom Images.
Configuring Training Parameters
Data is obtained from an OBS bucket or dataset for model training. The training output can also be stored in an OBS bucket. When creating a training job, you can configure parameters such as input, output, hyperparameters, and environment variables by referring to Table 4.
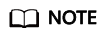
The input, output, and hyperparameter parameters of a training job vary depending on the algorithm type selected during training job creation. If a parameter value is dimmed, the parameter has been configured in the algorithm code and cannot be modified.
Parameter |
Sub-Parameter |
Description |
---|---|---|
Input |
Parameter name |
The algorithm code reads the training input data based on the input parameter name. The recommended value is data_url. The training input parameters must match the input parameters of the selected algorithm. For details, see Table 4. |
Dataset |
Click Dataset and select the target dataset and its version in the ModelArts dataset list. When the training job is started, ModelArts automatically downloads the data in the input path to the training container.
NOTE:
ModelArts data management is being upgraded and is invisible to users who have not used data management. It is recommended that new users store their training data in OBS buckets. |
|
Data path |
Click Data path and select the storage path to the training input data from an OBS bucket. Files must not exceed 10 GB in total size, 1,000 in number, or 1 GB per file. When the training job is started, ModelArts automatically downloads the data in the input path to the training container. |
|
Obtained from |
The following uses training input data_path as an example.
|
|
Output |
Parameter name |
The algorithm code reads the training output data based on the output parameter name. The recommended value is train_url. The training output parameters must match the output parameters of the selected algorithm. For details, see Table 5. |
Data path |
Click Data path and select the storage path for the training output data from an OBS bucket. Files must not exceed 1 GB in total size, 128 in number, or 128 MB per file. During training, the system automatically synchronizes files from the local code directory of the training container to the data path.
NOTE:
The data path can only be an OBS path. To prevent any issues with data storage, choose an empty directory as the data path. |
|
Obtained from |
The following uses the training output train_url as an example.
|
|
Predownload |
Indicates whether to pre-download the files in the output directory to a local directory.
|
|
Hyperparameter |
N/A |
Used for training tuning. This parameter is determined by the selected algorithm. If hyperparameters have been defined in the algorithm, all hyperparameters in the algorithm are displayed. Hyperparameters can be modified and deleted. The status depends on the hyperparameter constraint settings in the algorithm. For details, see Table 6. To import hyperparameters in batches, click Upload. You will need to fill in the hyperparameters based on the provided template. The total number of hyperparameters should not exceed 100, or the import will fail.
NOTE:
To ensure data security, do not enter sensitive information, such as plaintext passwords. |
Environment Variable |
N/A |
Add environment variables based on service requirements. For details about the environment variables preset in the training container, see Managing Environment Variables of a Training Container. To import environment variables in batches, click Upload. You will need to fill in the environment variables based on the provided template. The total number of environment variables should not exceed 100, or the import will fail.
NOTE:
To ensure data security, do not enter sensitive information, such as plaintext passwords. |
Auto Restart |
N/A |
Once this feature is enabled, you can set the number of restarts and whether to enable Unconditional auto restart. After you enable auto restart, ModelArts will handle any exceptions caused by environmental issues during a training job. It will either automatically handle the exception or isolate the faulty node and then restart the job, which helps to increase the success rate of the training. To avoid losing training progress and make full use of compute power, ensure that your code logic supports resumable training before enabling this function. For details, see Resumable Training. The value ranges from 1 to 128. The default value is 3. The value cannot be changed once the training job is created. Set this parameter based on your needs. If Unconditional auto restart is selected, the training job will be restarted unconditionally once the system detects a training exception. To prevent invalid restarts, it supports a maximum of three consecutive unconditional restarts. ModelArts continuously monitors job processes to detect suspension and optimize resource usage. When Restart Upon Suspension is enabled, suspended jobs can be automatically restarted at the process level. However, ModelArts does not verify code logic, and suspension detection is periodic, which may result in false reports. By enabling this feature, you acknowledge the possibility of false positives. To prevent unnecessary restarts, ModelArts limits consecutive restarts to three. If auto restart is triggered during training, the system records the restart information. You can check the fault recovery details on the training job details page. For details, see Training Job Rescheduling. |
Configuring a Public Resource Pool
Parameter |
Description |
---|---|
Resource Pool |
Select Public resource pool. |
Resource Type |
Select the resource type required for training. This parameter is mandatory. If a resource type has been defined in the training code, select a proper resource type based on algorithm constraints. For example, if the resource type defined in the training code is CPU and you select other types, the training fails. If some resource types are invisible or unavailable for selection, they are not supported. |
Specifications |
Select the required resource specifications based on the resource type. If Data path is selected for Input, you can click Check Input Size on the right to ensure the storage is larger than the input data size.
NOTICE:
The resource flavor GPU:n*tnt004 (n indicates a specific number) does not support multi-process training. |
Compute Nodes |
Select the number of instances as required. The default value is 1.
|
Persistent Log Saving |
If you select CPU or GPU flavors, Persistent Log Saving is available for you to configure.
|
Job Log Path |
When enabling Persistent Log Saving, select an empty OBS directory for Job Log Path to store log files generated by the training job. Ensure that you have read and write permissions to the selected OBS directory. |
Event Notification |
Indicates whether to enable event notification.
NOTE:
|
Auto Stop |
When using paid resources, you can determine whether to enable auto stop.
|
training_ssh_configure_nodes |
Whether to enable password-free SSH mutual trust between nodes.
|
Configuring a Dedicated Resource Pool
Parameter |
Description |
---|---|
Resource Pool |
Select a dedicated resource pool. If you select a dedicated resource pool, you can view the status, node specifications, number of idle/fragmented nodes, number of available/total nodes, and number of cards of the resource pool. Hover over View in the Idle/Fragmented Nodes column to check fragment details and check whether the resource pool meets the training requirements. |
Specifications |
Select the required resource specifications based on the resource type. If Data path is selected for Input, you can click Check Input Size on the right to ensure the storage is larger than the input data size.
NOTICE:
The resource flavor GPU:n*tnt004 (n indicates a specific number) does not support multi-process training. |
Compute Nodes |
Select the number of instances as required. The default value is 1.
|
Job Priority |
When using a dedicated resource pool, you can set the priority of the training job. The value ranges from 1 to 3. The default priority is 1, and the highest priority is 3.
|
SFS Turbo |
When ModelArts and SFS Turbo are directly connected, multiple SFS Turbo file systems can be mounted to a training job to store training data. Click Add Mount Configuration and set the following parameters:
NOTE:
|
Persistent Log Saving |
If you select CPU or GPU flavors, Persistent Log Saving is available for you to configure.
|
Job Log Path |
When enabling Persistent Log Saving, select an empty OBS directory for Job Log Path to store log files generated by the training job. Ensure that you have read and write permissions to the selected OBS directory. |
Event Notification |
Indicates whether to enable event notification.
NOTE:
|
Auto Stop |
When using paid resources, you can determine whether to enable auto stop.
|
training_ssh_configure_nodes |
Whether to enable password-free SSH mutual trust between nodes.
|
(Optional) Selecting a Training Mode
Select a training mode when you use a preset MindSpore engine and Ascend resources. ModelArts provides three training modes for you to select. You can obtain different diagnosis information based on the actual scenario.
- Common mode: It is the default training scenario.
- High performance mode: In this mode, certain O&M functions will be adjusted or even disabled to accelerate the running speed, but this will deteriorate fault locating. This mode is suitable for stable networks requiring high performance.
- Fault diagnosis mode: In this mode, certain O&M functions will be enabled or adjusted to collect more information for locating faults. This mode provides fault diagnosis. You can select a diagnosis type as required.
(Optional) Adding Tags
If you want to manage training jobs by group using tags, select Configure Now for Advanced Configuration to set tags for training jobs. For details about how to use tags, see Using TMS Tags to Manage Resources by Group.
Follow-Up Operations
After parameter setting for creating a training job, click Submit. On the Confirm dialog box, click OK.
A training job runs for a period of time. You can go to the training job list to view the basic information about the training job.
- In the training job list, Status of a newly created training job is Pending.
- When the status of a training job changes to Completed, the training job is finished, and the generated model is stored in the corresponding output path.
- If the status is Failed or Abnormal, click the job name to go to the job details page and view logs for troubleshooting.
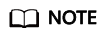
You are billed for the resources you choose when your training job runs.
Feedback
Was this page helpful?
Provide feedbackThank you very much for your feedback. We will continue working to improve the documentation.See the reply and handling status in My Cloud VOC.
For any further questions, feel free to contact us through the chatbot.
Chatbot