Preparing Data
Before using ModelArts ExeML to build a model, upload data to an OBS bucket. The OBS bucket and ModelArts must be in the same region.
Uploading Data to OBS
This operation uses the OBS console to upload data.
Perform the following operations to import data to the dataset for model training and building.
- Log in to OBS Console and create a bucket in the same region as ModelArts. If an available bucket exists, ensure that the OBS bucket and ModelArts are in the same region.
- Upload a file to the OBS bucket. If you have a large amount of data, use OBS Browser+ to upload data or folders. The uploaded data must meet the dataset requirements of the ExeML project.
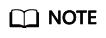
Upload data from unencrypted buckets. Otherwise, training will fail because data cannot be decrypted.
Requirements on Datasets
- The name of files in a dataset cannot contain Chinese characters, plus signs (+), spaces, or tabs.
- Ensure that no damaged image exists. The supported image formats include JPG, JPEG, BMP, and PNG.
- Do not store data of different projects in the same dataset.
- To ensure the prediction accuracy of models, the training samples must be similar to the actual application scenarios.
- To ensure the generalization capability of models, datasets should cover all possible scenarios.
- In an object detection dataset, if the coordinates of the bounding box exceed the boundaries of an image, the image cannot be identified as a labeled image.
Requirements for Files Uploaded to OBS
- If you do not need to upload training data in advance, create an empty folder to store files generated in the future, for example, /bucketName/data-cat.
- If you need to upload images to be labeled in advance, create an empty folder and save the images in the folder. An example of the image directory structure is /bucketName/data-cat/cat.jpg.
- If you want to upload labeled images to the OBS bucket, upload them according to the following specifications:
- The dataset for object detection requires storing labeled objects and their label files (in one-to-one relationship with the labeled objects) in the same directory. For example, if the name of the labeled object is IMG_20180919_114745.jpg, the name of the label file must be IMG_20180919_114745.xml.
The label files for object detection must be in PASCAL VOC format. For details about the format, see Table 1.
Example of data files:├─<dataset-import-path> │ IMG_20180919_114732.jpg │ IMG_20180919_114732.xml │ IMG_20180919_114745.jpg │ IMG_20180919_114745.xml │ IMG_20180919_114945.jpg │ IMG_20180919_114945.xml
- Images in JPG, JPEG, PNG, and BMP formats are supported. When uploading images on the OBS console, ensure that the size of an image does not exceed 5 MB and the total size of images to be uploaded in one attempt does not exceed 8 MB. If the data volume is large, use OBS Browser+ to upload images.
- A label name can contain a maximum of 32 characters, including letters, digits, hyphens (-), and underscores (_).
Table 1 PASCAL VOC format description Field
Mandatory
Description
folder
Yes
Directory where the data source is located
filename
Yes
Name of the file to be labeled
size
Yes
Image pixel
- width: image width. This parameter is mandatory.
- height: image height. This parameter is mandatory.
- depth: number of image channels. This parameter is mandatory.
segmented
Yes
Segmented or not
object
Yes
Object detection information. Multiple object{} functions are generated for multiple objects.
- name: class of the labeled object. This parameter is mandatory.
- pose: shooting angle of the labeled object. This parameter is mandatory.
- truncated: whether the labeled object is truncated (0 indicates that the object is not truncated). This parameter is mandatory.
- occluded: whether the labeled object is occluded (0 indicates that the object is not occluded). This parameter is mandatory.
- difficult: whether the labeled object is difficult to identify (0 indicates that the object is easy to identify). This parameter is mandatory.
- confidence: confidence score of the labeled object. The value range is 0 to 1. This parameter is optional.
- bndbox: bounding box type. This parameter is mandatory. For details about the possible values, see Table 2.
Table 2 Description of bounding box types type
Shape
Labeling Information
bndbox
Rectangle
Coordinates of the upper left and lower right points
<xmin>100<xmin>
<ymin>100<ymin>
<xmax>200<xmax>
<ymax>200<ymax>
Example of the label file in KITTI format:<annotation> <folder>test_data</folder> <filename>260730932.jpg</filename> <size> <width>767</width> <height>959</height> <depth>3</depth> </size> <segmented>0</segmented> <object> <name>bag</name> <pose>Unspecified</pose> <truncated>0</truncated> <occluded>0</occluded> <difficult>0</difficult> <bndbox> <xmin>108</xmin> <ymin>101</ymin> <xmax>251</xmax> <ymax>238</ymax> </bndbox> </object> </annotation>
- The dataset for object detection requires storing labeled objects and their label files (in one-to-one relationship with the labeled objects) in the same directory. For example, if the name of the labeled object is IMG_20180919_114745.jpg, the name of the label file must be IMG_20180919_114745.xml.
Feedback
Was this page helpful?
Provide feedbackThank you very much for your feedback. We will continue working to improve the documentation.See the reply and handling status in My Cloud VOC.
For any further questions, feel free to contact us through the chatbot.
Chatbot