Creating a Service Deployment Phase
Description
This phase integrates capabilities of ModelArts service management to enable service deployment and update in a workflow. The application scenarios are as follows:
- Deploying a model as a web service
- Updating an existing service (gray update supported)
Parameter Overview
You can use ServiceStep to create a service deployment phase. The following is an example of defining a ServiceStep.
Parameter |
Description |
Mandatory |
Data Type |
---|---|---|---|
name |
Name of a service deployment phase. The name contains a maximum of 64 characters, including only letters, digits, underscores (_), and hyphens (-). It must start with a letter and must be unique in a workflow. |
Yes |
str |
inputs |
Inputs of the service deployment phase. |
No |
ServiceInput or ServiceInput list |
outputs |
Outputs of the service deployment phase. |
Yes |
ServiceOutput or ServiceOutput list |
title |
Title for frontend display. |
No |
str |
description |
Description of the service deployment phase. |
No |
str |
policy |
Phase execution policy. |
No |
StepPolicy |
depend_steps |
Dependent phases. |
No |
Step or step list |
Parameter |
Description |
Mandatory |
Data Type |
---|---|---|---|
name |
Input name of the service deployment phase. The name can contain a maximum of 64 characters, including only letters, digits, underscores (_), and hyphens (-), and must start with a letter. The input name of a step must be unique. |
Yes |
str |
data |
Input data object of the service deployment phase. |
Yes |
Model list or service object. Currently, only ServiceInputPlaceholder, ServiceData, and ServiceUpdatePlaceholder are supported. |
Parameter |
Description |
Mandatory |
Data Type |
---|---|---|---|
name |
Output name of the service deployment phase. The name can contain a maximum of 64 characters, including only letters, digits, underscores (_), and hyphens (-), and must start with a letter. The output name of a step must be unique. |
Yes |
str |
service_config |
Configurations for service deployment. |
Yes |
ServiceConfig |
Table 4 ServiceConfig
Parameter |
Description |
Mandatory |
Data Type |
---|---|---|---|
infer_type |
Inference mode. The value can be real-time, batch, or edge. The default value is real-time.
|
Yes |
str |
service_name |
Service name. Enter 1 to 64 characters. Only letters, digits, hyphens (-), and underscores (_) are allowed.
NOTE:
If you do not specify this parameter, the default service name is generated automatically. |
No |
str, Placeholder |
description |
Service description, which contains a maximum of 100 characters. By default, this parameter is left blank. |
No |
str |
vpc_id |
ID of the VPC to which a real-time service instance is deployed. By default, this parameter is left blank. In this case, ModelArts allocates a dedicated VPC to each user, and users are isolated from each other. To access other service components in the VPC of the service instance, set this parameter to the ID of the corresponding VPC. Once a VPC is configured, it cannot be modified. If both vpc_id and cluster_id are configured, only the dedicated resource pool takes effect. |
No |
str |
subnet_network_id |
ID of a subnet. By default, this parameter is left blank. This parameter is mandatory when vpc_id is configured. Enter the network ID displayed in the subnet details on the VPC management console. A subnet provides dedicated network resources that are isolated from other networks. |
No |
str |
security_group_id |
Security group. By default, this parameter is left blank. This parameter is mandatory when vpc_id is configured. A security group is a virtual firewall that provides secure network access control policies for service instances. A security group must contain at least one inbound rule to permit the requests whose protocol is TCP, source address is 0.0.0.0/0, and port number is 8080. |
No |
str |
cluster_id |
ID of a dedicated resource pool. By default, this parameter is left blank, indicating that no dedicated resource pool is used. When using a dedicated resource pool to deploy services, ensure that the cluster is running properly. After this parameter is configured, the network configuration of the cluster is used, and the vpc_id parameter does not take effect. If both this parameter and cluster_id in real-time config are configured, cluster_id in real-time config is preferentially used. |
No |
str |
additional_properties |
Additional configurations. |
No |
dict |
apps |
Whether to enable application authentication for service deployment. Multiple application names can be entered. |
No |
str, Placeholder, list |
envs |
Environment variables. |
No |
dict |
Example:
example = ServiceConfig() # This object is used in the output of the service deployment phase.
If there is no special requirement, use the default values.
Examples
There are three scenarios:
- Deploying a real-time service
- Modifying a real-time service
- Getting the inference address from the service deployment phase
Deploying a Real-Time Service
import modelarts.workflow as wf # Use ServiceStep to define a service deployment phase and specify a model for service deployment. # Define model name parameters. model_name = wf.Placeholder(name="placeholder_name", placeholder_type=wf.PlaceholderType.STR) service_step = wf.steps.ServiceStep( name="service_step", # Name of the service deployment phase. The name contains a maximum of 64 characters, including only letters, digits, underscores (_), and hyphens (-). It must start with a letter and must be unique in a workflow. title="Deploying a Service", # Title inputs=wf.steps.ServiceInput(name="si_service_ph", data=wf.data.ServiceInputPlaceholder(name="si_placeholder1", # Restrictions on the model name: Only the model name specified here can be used in the running state; use the same model name as model_name of the model registration phase. model_name=model_name)),# ServiceStep inputs outputs=wf.steps.ServiceOutput(name="service_output") # ServiceStep outputs ) workflow = wf.Workflow( name="service-step-demo", desc="this is a demo workflow", steps=[service_step] )
Modifying a Real-Time Service
Scenario: When you use a new model version to update an existing service, ensure that the name of the new model version is the same as that of the deployed service.
import modelarts.workflow as wf # Use ServiceStep to define a service deployment phase and specify a model for service update. # Define model name parameters. model_name = wf.Placeholder(name="placeholder_name", placeholder_type=wf.PlaceholderType.STR) # Define a service object. service = wf.data.ServiceUpdatePlaceholder(name="placeholder_name") service_step = wf.steps.ServiceStep( name="service_step", # Name of the service deployment phase. The name contains a maximum of 64 characters, including only letters, digits, underscores (_), and hyphens (-). It must start with a letter and must be unique in a workflow. title="Service Update", # Title inputs=[wf.steps.ServiceInput(name="si2", data=wf.data.ServiceInputPlaceholder(name="si_placeholder2", # Restrictions on the model name: Only the model name specified here can be used in the running state. model_name=model_name)), wf.steps.ServiceInput(name="si_service_data", data=service) # ServiceStep inputs are configured when the workflow is running. You can also use wf.data.ServiceData(service_id="fake_service") for the data field. ], # ServiceStep inputs outputs=wf.steps.ServiceOutput(name="service_output") # ServiceStep outputs ) workflow = wf.Workflow( name="service-step-demo", desc="this is a demo workflow", steps=[service_step] )
Getting the Inference Address from the Service Deployment Phase
The service deployment phase supports the output of the inference address. You can use the get_output_variable("access_address") method to obtain the output and use it in subsequent phases.
- For services deployed in the public resource pool, you can use access_address to obtain the inference address registered on the public network from the output.
- For services deployed in a dedicated resource pool, you can get the internal inference address from the output using cluster_inner_access_address, in addition to the public inference address. The internal address can only be accessed by other inference services.
import modelarts.workflow as wf # Define model name parameters. sub_model_name = wf.Placeholder(name="si_placeholder1", placeholder_type=wf.PlaceholderType.STR) sub_service_step = wf.steps.ServiceStep( name="sub_service_step", # Name of the service deployment phase. The name contains a maximum of 64 characters, including only letters, digits, underscores (_), and hyphens (-). It must start with a letter and must be unique in a workflow. title="Subservice", # Title inputs=wf.steps.ServiceInput( name="si_service_ph", data=wf.data.ServiceInputPlaceholder(name="si_placeholder1", model_name=sub_model_name) ),# ServiceStep inputs outputs=wf.steps.ServiceOutput(name="service_output") # ServiceStep outputs ) main_model_name = wf.Placeholder(name="si_placeholder2", placeholder_type=wf.PlaceholderType.STR) # Obtain the inference address output by the subservice and transfer the address to the main service through envs. main_service_config = wf.steps.ServiceConfig( infer_type="real-time", envs={"infer_address": sub_service_step.outputs["service_output"].get_output_variable("access_address")} # Obtain the inference address output by the subservice and transfer the address to the main service through envs. ) main_service_step = wf.steps.ServiceStep( name="main_service_step", # Name of the service deployment phase. The name contains a maximum of 64 characters, including only letters, digits, underscores (_), and hyphens (-). It must start with a letter and must be unique in a workflow. title="Main service", # Title inputs=wf.steps.ServiceInput( name="si_service_ph", data=wf.data.ServiceInputPlaceholder(name="si_placeholder2", model_name=main_model_name) ),# ServiceStep inputs outputs=wf.steps.ServiceOutput(name="service_output", service_config=main_service_config), # ServiceStep outputs depend_steps=sub_service_step ) workflow = wf.Workflow( name="service-step-demo", desc="this is a demo workflow", steps=[sub_service_step, main_service_step] )
Configuring Information for Deploying a Synchronous Service
After the service deployment phase is started in the development state (usually a notebook instance), configure the information based on the following format in the logs.
- On the ModelArts console, choose Development Workspace > Workflow from the navigation pane.
- Configure the information after the service deployment phase is started. After the configuration, click Next.
Configuring Information for Deploying an Asynchronous Service
- On the ModelArts console, choose Workflow from the navigation pane.
- Configure the information after the service deployment phase is started. Select an asynchronous inference AI application and a version, and configure service startup parameters. After the configuration, click Next.
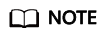
After you select the required AI application and version, the system automatically matches the service startup parameters.
Feedback
Was this page helpful?
Provide feedbackThank you very much for your feedback. We will continue working to improve the documentation.See the reply and handling status in My Cloud VOC.
For any further questions, feel free to contact us through the chatbot.
Chatbot