Creating a Training Job
Sample Code
In ModelArts notebook, you do not need to enter authentication parameters for session authentication. For details about session authentication of other development environments, see Session Authentication.
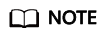
ModelArts SDK cannot be used to create training jobs using algorithms subscribed to in AI Gallery.
- Example 1: Create a training job using a common AI engine.
If both framework_type and framework_version are specified in estimator, a training job will be created using a common AI engine.
from modelarts.session import Session from modelarts.train_params import TrainingFiles from modelarts.train_params import OutputData from modelarts.train_params import InputData from modelarts.estimatorV2 import Estimator session = Session() # Parameters received in the training script (set based on the site requirements): parameters = [{"name": "mod", "value":"gpu"}, {"name": "epoc_num", "value":2}] estimator = Estimator(session=session, training_files=TrainingFiles(code_dir= "obs://bucket_name/code_dir/", boot_file="boot_file.py"), outputs=[OutputData(obs_path="obs://bucket_name/output/", name="output_dir")], parameters=parameters, framework_type='PyTorch', # Common AI engine framework_version='PyTorch-1.4.0-python3.6', # Version of the AI engine train_instance_type="modelarts.p3.large.public", train_instance_count=1, log_url="obs://bucket_name/log/", env_variables={"USER_ENV_VAR": "customize environment variable"}, working_dir="/home/ma-user/modelarts/user-job-dir", local_code_dir="/home/ma-user/modelarts/user-job-dir", job_description='This is an image net train job') job_instance = estimator.fit(inputs=[InputData(obs_path="obs://bucket_name/input/", name="data_url")], job_name="job_name_1")
- Example 2: Create a training job using a custom image.
If both user_image_url and user_command are specified in estimator, a training job will be created using a custom image and started using a custom boot command.
from modelarts.session import Session from modelarts.train_params import TrainingFiles from modelarts.train_params import OutputData from modelarts.train_params import InputData from modelarts.estimatorV2 import Estimator session = Session() # Parameters received in the training script (set based on the site requirements): parameters = [{"name": "mod", "value":"gpu"}, {"name": "epoc_num", "value":2}] estimator = Estimator(session=session, training_files=TrainingFiles(code_dir= "obs://bucket_name/code_dir/", boot_file="boot_file.py"), outputs=[OutputData(obs_path="obs://bucket_name/output/", name="output_dir")], parameters=parameters, user_image_url="sdk-test/pytorch1_4:1.0.1", # URL of the custom image user_command="/home/ma-user/anaconda3/envs/PyTorch-1.4/bin/python /home/ma-user/modelarts/user-job-dir/train/test-pytorch.py", # Custom boot command train_instance_type="modelarts.p3.large.public", train_instance_count=1, log_url="obs://bucket_name/log/", env_variables={"USER_ENV_VAR": "customize environment variable"}, working_dir="/home/ma-user/modelarts/user-job-dir", local_code_dir="/home/ma-user/modelarts/user-job-dir", job_description='This is an image net train job') job_instance = estimator.fit(inputs=[InputData(obs_path="obs://bucket_name/input/", name="data_url")], job_name="job_name_2")
- Example 3: Creating a training job in a dedicated resource pool
from modelarts.session import Session from modelarts.train_params import TrainingFiles from modelarts.train_params import OutputData from modelarts.train_params import InputData from modelarts.estimatorV2 import Estimator session = Session() # Parameters received in the training script (set based on the site requirements): parameters = [{"name": "mod", "value":"gpu"}, {"name": "epoc_num", "value":2}] estimator = Estimator(session=session, training_files=TrainingFiles(code_dir= "obs://bucket_name/code_dir/", boot_file="boot_file.py"), outputs=[OutputData(obs_path="obs://bucket_name/output/", name="output_dir")], parameters=parameters, framework_type='PyTorch', framework_version='PyTorch-1.4.0-python3.6', pool_id="your pool id", # Dedicated resource pool ID train_instance_type="modelarts.pool.visual.xlarge", # VM flavor of the dedicated pool train_instance_count=1, log_url="obs://bucket_name/log/", env_variables={"USER_ENV_VAR": "customize environment variable"}, working_dir="/home/ma-user/modelarts/user-job-dir", local_code_dir="/home/ma-user/modelarts/user-job-dir", job_description='This is an image net train job') job_instance = estimator.fit(inputs=[InputData(obs_path="obs://bucket_name/input/", name="data_url")], job_name="job_name_3")
- Example 4: Create a training job using a dataset.
from modelarts.session import Session from modelarts.train_params import TrainingFiles from modelarts.train_params import OutputData from modelarts.train_params import InputData from modelarts.estimatorV2 import Estimator session = Session() # Parameters received in the training script (set based on the site requirements): parameters = [{"name": "model_name", "value":"s"}, {"name": "batch-size", "value": 32}, {"name": "epochs", "value":100}, {"name": "img-size", "value":"640,640"} ] estimator = Estimator(session=session, training_files=TrainingFiles(code_dir= "obs://bucket_name/code_dir/", boot_file="boot_file.py"), outputs=[OutputData(obs_path="obs://bucket_name/output/", name="output_dir")], parameters=parameters, framework_type='PyTorch', # Common AI engine framework_version='PyTorch-1.4.0-python3.6', # Version of the AI engine train_instance_type="modelarts.p3.large.public", train_instance_count=1, log_url="obs://bucket_name/log/", working_dir="/home/ma-user/modelarts/user-job-dir", local_code_dir="/home/ma-user/modelarts/user-job-dir", job_description='This is an image net train job') job_instance = estimator.fit(dataset_id="your dataset id", dataset_version_id="your dataset version id", job_name="job_name_5")
Parameters
Parameter |
Mandatory |
Type |
Description |
---|---|---|---|
session |
Yes |
Object |
Session object. For details about the initialization method, see Session Authentication. |
training_files |
No |
TrainingFiles Object |
Path to the training script in OBS. For details, see Table 2. |
outputs |
No |
Array of OutputData objects |
Training output path. For details, see Table 3. |
parameters |
No |
JSON Array |
Running parameters of a training job. The format is as follows: [{"name":"your name", "value": "your value"}]. The value can be a string or an integer. |
train_instance_type |
Yes |
String |
Resource flavor selected for a training job. For details, see Obtaining Resource Flavors. |
train_instance_count |
Yes |
Int |
Number of compute nodes in a training job |
framework_type |
No |
String |
Engine type selected for a training job. For details, see Obtaining Engine Types. |
framework_version |
No |
String |
Engine version selected for a training job. For details, see Obtaining Engine Types. |
user_image_url |
No |
String |
SWR URL of the custom image used by a training job |
user_command |
No |
String |
Command for starting a training job created using a custom image |
log_url |
No |
String |
OBS path for storing training job logs, for example, obs://xx/yy/zz/ |
local_code_dir |
No |
String |
Local directory to the training container to which the algorithm code directory is downloaded. Note:
|
working_dir |
No |
String |
Work directory where an algorithm is executed. Note that this parameter does not take effect in v1 compatibility mode. |
job_description |
No |
String |
Description of a training job |
volumes |
No |
JSON Array |
Information of the disks attached for a training job in the following example format: [{ "nfs": { "local_path": "/xx/yy/zz", "read_only": False, "nfs_server_path": "xxx.xxx.xxx.xxx:/" } }] |
env_variables |
No |
Dict |
Environment variables of a training job |
pool_id |
No |
String |
ID of the resource pool for a training job. To obtain the ID, do as follows: Log in to the ModelArts management console, choose Dedicated Resource Pools in the navigation pane on the left, and view the resource pool ID in the dedicated resource pool list. |
Parameter |
Mandatory |
Type |
Description |
---|---|---|---|
code_dir |
Yes |
String |
Code directory of a training job, which is an OBS path and must start with obs:/, for example, obs://xx/yy/ |
boot_file |
Yes |
String |
Boot file of a training job, which must be stored in the code directory. You can enter a relative path, for example, boot_file.py, or an absolute path, for example, obs://xx/yy/boot_file.py. |
Parameter |
Mandatory |
Type |
Description |
---|---|---|---|
obs_path |
Yes |
String |
OBS path to which data is exported |
name |
Yes |
String |
Keyword parameter name of the output data, for example, output_dir |
Parameter |
Mandatory |
Type |
Description |
---|---|---|---|
inputs |
No |
Array of InputData Object |
Input data of a training job stored in OBS Either inputs or dataset_id/dataset_version_id can be configured. |
wait |
No |
Boolean |
Whether to wait for the completion of a training job. It defaults to False. |
job_name |
No |
String |
Name of a training job |
show_log |
No |
Boolean |
Whether to output training job logs after a job is submitted. It defaults to False. |
dataset_id |
No |
String |
Dataset ID of a training job. For details, see Data Management. This parameter must be used with dataset_version_id, but cannot be used with inputs. |
dataset_version_id |
No |
String |
Dataset version ID of a training job. For details, see Data Management. This parameter must be used with dataset_id, but cannot be used with inputs. |
Parameter |
Mandatory |
Type |
Description |
---|---|---|---|
obs_path |
Yes |
String |
OBS path to the dataset required by a training job, for example, obs://xx/yy/ |
name |
Yes |
String |
Keyword parameter name of the input data, for example, data_url. |
Parameter |
Type |
Description |
---|---|---|
TrainingJob |
Object |
Training object, which contains attributes such as job_id. When you perform operations on a training job, for example, obtain information of, update, or delete a training job, you can use job_instance.job_id to obtain the ID of the training job. |
Parameter |
Type |
Description |
---|---|---|
error_msg |
String |
Error message when calling an API failed. This parameter is unavailable if an API is successfully called. |
error_code |
String |
Error code when calling an API failed. For details, see "Error Codes" in ModelArts API Reference. This parameter is unavailable if an API is successfully called. |
error_solution |
String |
Solution to an API calling failure. This parameter is unavailable if an API is successfully called. |
Feedback
Was this page helpful?
Provide feedbackThank you very much for your feedback. We will continue working to improve the documentation.